the Creative Commons Attribution 4.0 License.
the Creative Commons Attribution 4.0 License.
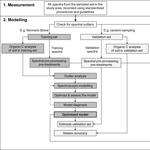
Proximal sensing for soil carbon accounting
Jacqueline R. England
Raphael A. Viscarra Rossel
Maintaining or increasing soil organic carbon (C) is vital for securing food production and for mitigating greenhouse gas (GHG) emissions, climate change, and land degradation. Some land management practices in cropping, grazing, horticultural, and mixed farming systems can be used to increase organic C in soil, but to assess their effectiveness, we need accurate and cost-efficient methods for measuring and monitoring the change. To determine the stock of organic C in soil, one requires measurements of soil organic C concentration, bulk density, and gravel content, but using conventional laboratory-based analytical methods is expensive. Our aim here is to review the current state of proximal sensing for the development of new soil C accounting methods for emissions reporting and in emissions reduction schemes. We evaluated sensing techniques in terms of their rapidity, cost, accuracy, safety, readiness, and their state of development. The most suitable method for measuring soil organic C concentrations appears to be visible–near-infrared (vis–NIR) spectroscopy and, for bulk density, active gamma-ray attenuation. Sensors for measuring gravel have not been developed, but an interim solution with rapid wet sieving and automated measurement appears useful. Field-deployable, multi-sensor systems are needed for cost-efficient soil C accounting. Proximal sensing can be used for soil organic C accounting, but the methods need to be standardized and procedural guidelines need to be developed to ensure proficient measurement and accurate reporting and verification. These are particularly important if the schemes use financial incentives for landholders to adopt management practices to sequester soil organic C. We list and discuss requirements for developing new soil C accounting methods based on proximal sensing, including requirements for recording, verification, and auditing.
- Article
(394 KB) - Full-text XML
- BibTeX
- EndNote
Soil is the most abundant terrestrial store of organic carbon (C) (Batjes, 1996). Soil organic C is an indicator of soil quality because it affects nutrient cycling, aggregate stability, structure, water infiltration, and vulnerability to erosion (Lal, 2013; Tiessen et al., 1994). Management of soil organic C is central to maintaining soil health, ensuring food security, and mitigating climate change (Lal, 2004, 2016b). This is why stabilizing or increasing the stocks of organic C in soil, through the identification and implementation of locally appropriate agronomic and environmental management practices, has become a political imperative (ITPS, 2015).
Maintaining or increasing soil organic C stocks is essential for addressing land degradation, which was recognized under the United Nations Sustainable Development Goal 15, i.e. the sustainable development of life on land (United Nations, 2015). Used in concert with data on land cover and land productivity, soil organic C stock will be the first metric used to quantify Indicator 15.3.1, the “Proportion of land that is degraded over total land area” (Decision 22/COP.11; UNCCD, 2013). The United Nations Convention to Combat Desertification (UNCCD) will use soil organic C stock as one of its indicators to monitor progress towards achieving land degradation neutrality (Orr et al., 2017).
Sequestration of soil organic C has been considered as a possible solution to mitigate climate change (Lal, 2016a). Both policy makers and scientists expect there is significant potential for sequestration in agricultural soils due to the large historical losses experienced by agroecosystems (Cole et al., 1997). Studies over several decades have demonstrated that improved land use and management can help to sequester organic C in soil and to reduce greenhouse gas (GHG) emissions (Ogle et al., 2005; Poeplau and Don, 2015; Smith et al., 2008). This opportunity was the motivation behind the “4 pour 1000” initiative, launched at the COP21 meeting in Paris, which aims to increase global soil organic C stocks by 4 parts per 1000 (or 0.4 %) per year as compensation for global GHG emissions from anthropogenic sources. Engagement in “4 pour 1000” involves stakeholders voluntarily committing to implementing farming practices that maintain or enhance organic C stocks in agricultural soil and to preserving C-rich soil (Lal, 2016a). In addition to increasing soil organic C, the initiative provides an opportunity to implement transparent and credible protocols and methods for C accounting, monitoring, reporting, and verification that are compatible with national GHG inventory procedures (Chambers et al., 2016).
Although there is good potential for changes in agricultural land use and management to sequester soil organic C (Machmuller et al., 2015), their implementation requires efficient new methods for measurement and monitoring of C stocks. To determine baselines and to assess the success of management practices for sequestering soil organic C, the variability in soil organic C stock needs to be quantified in both space (laterally across the landscape and vertically down the soil profile) and time. Changes in soil organic C stock that are due to changes in land use and management or climate occur slowly (e.g. compared to changes in biomass C) and must be measured over periods longer than 5 to 10 years (Smith, 2004). Changes are also likely to be small relative to the C stock that is present in the soil, which is variable. New measurement methods are also needed to delineate the potential benefits and liabilities of establishing soil C projects and C accounting activities (IPCC, 2007). Methods for soil organic C sampling and analysis must be accurate, practical, inexpensive, and cost-efficient and when used for monitoring, they must consider the minimum detectable difference (Batjes and van Wesemael, 2015). Additionally, the new methods should accurately quantify the magnitude and uncertainty of soil C change that could be attained by introducing defined agricultural management practices (Paustian et al., 2016).
The quantification of soil organic C stock change requires the measurement of soil organic C concentration, bulk density, and gravel content over time. Conventional methods to measure soil organic C stocks involve field soil sampling, followed by sample preparation and laboratory analysis. For the determination of soil C concentration, dry combustion (Nelson and Sommers, 1996) is the benchmark method because of the vast experience acquired in using it and because of its accuracy. For bulk density, conventional measurements are made using the volumetric ring method or the clod method for soil containing a lot of rock fragments (Blake and Hartge, 1986). For gravel content, the conventional procedure involves manual separation of gravel from the fine-earth fraction (McKenzie et al., 2002). These methods are time-consuming and expensive, particularly for measuring at depth.
Sensors can provide rapid, accurate, inexpensive, and non-destructive measurements of soil organic C stocks and other soil properties. Their measurements are accurate and cost-efficient (Viscarra Rossel and Brus, 2018). While there are several reviews on the use of sensors for measuring soil organic C concentration (Bellon-Maurel and McBratney, 2011; Izaurralde et al., 2013; Reeves et al., 2012; Stenberg et al., 2010; Viscarra Rossel et al., 2011), few studies report sensors for measuring bulk density or gravel (Fouinat et al., 2017; Lobsey and Viscarra Rossel, 2016) or the integration of sensing for soil organic C accounting. Our objective here is to review the current state of proximal sensing for soil C accounting. Specifically, our aims were to review: (1) soil C accounting for emissions reporting and in emissions reduction schemes, (2) the current state of proximal sensing for measuring soil organic C stocks and monitoring its change and (3) the use of proximal sensors in the development of new soil organic C accounting methodologies.
The development of new technologies for soil organic C accounting concerns two areas of national GHG policy and reporting: national emissions reporting obligations under the United Nations Framework Convention on Climate Change (UNFCCC) and the Kyoto Protocol and domestic schemes that seek to reduce or offset emissions through a range of activities, including improved land management practices.
(Australian Government, 2014)(Australian Government, 2015)(Australian Government, 2018)Table 1Examples of methodologies for estimating change in soil organic C for mitigation actions related to changed management of agricultural land. Emissions Reduction Fund (ERF); Climate Change and Emissions Management Act (CCEMA); Alberta Offset System (AOS); Climate Policy Framework (CPF).
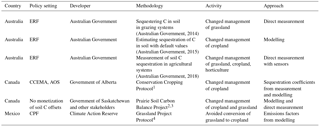
1 http://aep.alberta.ca/climate-change/guidelines-legislation/specified-gas-emitters-regulation/offset-credit-system-protocols.aspx (last access: 6 December 2017). 2 http://www.usask.ca/soilsncrops/conference-proceedings/previous_years/Files/cc2000/docs/posters/018_post.PDF (last access: 6 December 2017). 3 http://ssca.ca/images/new/PSCB.pdf (last access: 6 December 2017). 4 http://www.climateactionreserve.org/how/protocols/grassland/ (last access: 6 December 2017).
The UNFCCC, and later the Kyoto Protocol, set up a system of national communications and national inventory reporting to be compiled by parties and published by the UNFCCC. To estimate GHG emissions and to monitor changes in C stocks, including soil organic C, the International Panel on Climate Change (IPCC) developed a tiered methodology that relates data on land use and management activities to emissions and storage factors to estimate fluxes from the activities (IPCC, 2006). The three-tiered approach depends on the scale, capability, and availability of data. Where country-specific data are currently lacking, a global default (Tier 1) approach can be used. Tier 1 methods use default equations with data from globally available land cover classes and global defaults for reference soil organic C stocks, change factors, and emission factors. Tier 2 methods include nationally derived land cover classes and data for reference soil organic C stocks, change factors, and emission factors specific to local conditions. Tier 3 methods might include national data from the integration of ongoing ground-measurement programs, earth observation, and mechanistic models. Tier 2 and Tier 3 approaches are thought to produce estimates with reduced uncertainty.
National-scale soil monitoring networks can produce information on changes in soil organic C stocks relative to a baseline through repeated measurements across a defined network of sites over time. They can provide a set of observations that represent the variation in climate, soil, or land use management at a national scale (Batjes and van Wesemael, 2015). However, there are trade-offs between the ability to detect a change and the size of the network and the number of measurements required, which is directly related to cost (Conant and Paustian, 2002a). Conventional analytical methods for soil monitoring are likely to be cost-inefficient. Sensing, on the other hand, can be used to cost-efficiently measure soil organic C stocks and to estimate baselines for national inventory reporting and monitoring (Viscarra Rossel et al., 2014).
Accurate and cost-efficient methods to quantify changes in C stocks are also needed for a growing number of national and sub-national emissions reduction and C accounting and trading schemes that incorporate mitigation from soil organic C sequestration following changes in land management (ICAP, 2017). In this context, how to measure, report, and verify the impacts of mitigation actions is important for decision makers because access to financial payments depends on the ability to demonstrate the sequestration or emissions reductions that might be attained. To date, however, relatively few methodologies have been developed for quantifying a change in soil organic C stock from changes in land management. Those that have been developed are based on approaches that use either direct measurement or mechanistic modelling (Table 1). Under such schemes, endorsed methodologies set out the rules for estimating emissions reductions or C offsets from different activities. Proponents that change some permissible aspect of their land management, which leads to increases in net C stocks or reductions in emissions, can use these methods to earn payments. Information from these activities can then also contribute to national inventories.
For example, the Australian Government established the Emissions Reduction Fund (ERF) to encourage the adoption of management strategies that result in either the reduction of GHG emissions or the sequestration of atmospheric CO2. The ERF is enacted through the Carbon Credits (Carbon Farming Initiative) Act 2011 (CFI Act), and under it, carbon credits can be earned by anyone (e.g. landholders, businesses, and community groups) undertaking a project that aims to reduce emissions or sequester C (Australian Government, 2011). Projects must comply with approved methods (which are legislative instruments) that define the activities that are eligible to earn C credits and how the abatement is measured, verified, and reported. These methods1 must comply with the Offsets Integrity Standards described in the CFI Act, which require that any C abatement generated through the implementation of a method can be used to meet Australia's climate change targets under the Kyoto Protocol or other international agreements. The legislation ensures that only genuine emissions reductions are credited; that the methods used in the ERF are eligible, evidence-based (supported by relevant peer-reviewed science), measurable, verifiable, conservative, additional, and permanent (25 or 100 years); and that there is no leakage. Thamo and Pannell (2016) discuss these requirements and the challenges they pose for development of policy for soil C sequestration. Once a method is implemented by a proponent, it can be used to produce Australian Carbon Credit Units (ACCUs) and an offsets report that is then submitted to the Clean Energy Regulator. Proponents receive one ACCU for every tonne of emissions reduction. One ACCU corresponds to the sequestration or emission avoidance of 1 t of CO2 equivalent, which proponents can sell to generate income.
The Australian ERF currently has three soil C sequestration methods:
- i.
“Sequestering carbon in soils in grazing systems” (Australian Government, 2014) that aims to quantify changes in soil organic C stocks over time using conventional soil composite sampling and laboratory analysis;
- ii.
“Estimating sequestration of carbon in soil using default values” (Australian Government, 2015) that uses default values for the rates of soil C change from different activities, predicted with the Full Carbon Accounting Model (FullCAM), which is used in the Australian National Greenhouse Gas Inventory;
- iii.
“Measurement of Soil Carbon Sequestration in Agricultural Systems”, a new soil carbon method (Australian Government, 2018) legislated and made available on 25 January 2018. It increases the range of eligible activities to allow not only grazing businesses to participate but also cropping and horticultural businesses, it allows choice between different sampling designs to reduce sampling error (including the use of covariates and prior information to inform the design), and it allows the use of soil sensors to improve the accuracy and reduce the cost of measuring and monitoring soil carbon stocks. This is the first methodology in the world to legislate sensing for soil organic C accounting.
In Canada, the Government of Alberta amended the Climate Change and Emissions Management Act (CCEMA) in 2007 to require industries with substantial emissions to report and reduce their emissions to established targets. There are three options to meet the targets, one of which is emission offsets. The Alberta Offset System operates under a set of standards, known as the “Offset Quantification Protocols and Guidance Documents”. The development of protocols involves the inclusion of expert engagement, rigorous peer review, defensible scientific methodologies, and documented transparency. Under the Alberta Offset Scheme, the Conservation Cropping Protocol quantifies soil organic C change following changed land management from conventional cropping to conservation cropping (reduced tillage and summer fallow). The protocol uses Canada's National Emissions Tier 2 methodology, which developed C sequestration coefficients based on measuring and modelling local crop rotations, soil/landscape types, and inter-annual climate variation for geo-specific polygons in the national eco-stratification system. A second Canadian scheme in Saskatchewan, the Prairie Soil Carbon Balance (PSCB) Project was developed for quantifying and verifying the direct measurement of soil organic C changes in response to a shift from conventional tillage to no-till, direct-seeded cropping systems (McConkey et al., 2013). It was not designed to monetize soil carbon offsets but has been supported by farm groups with an interest in securing financial recognition for GHG mitigation. A regional monitoring project was established to measure the temporal change in soil C storage under agricultural cropland on the Canadian Prairies (Ellert et al., 2001). The sampling scheme reported in Ellert et al. (2001) for monitoring was designed to maximize the ability to detect changes in soil C over time by ensuring that exact sample locations can be relocated, limiting horizontal variability of soil C. This PSCB approach was subsequently used to assess sampling designs at contrasting scales in the USA (Conant and Paustian, 2002b).
3.1 Measuring soil organic C stocks
Effective accounting of changes in soil organic C stocks requires the measurement of the stocks and their uncertainty for a defined baseline and over the monitoring period. Internationally, the default method to determine soil organic C stock (Cs) for the accounting of change is to multiply measurements of soil organic C concentration, bulk density, and gravel content at a fixed depth of 0–30 cm and to report the stock as a mass of carbon per unit area in tonnes of organic C per hectare (IPCC, 1997):
where Cm is the mass of soil organic C in the soil (%), ρ is the soil bulk density (g cm−3), g is the gravel content (%), and d is the thickness of the layer (cm). Our definition of soil organic C used here extends that of the IPCC Guidelines (IPCC, 2006), which address the measurement of soil organic C in mineral soil in the 0–30 cm layer, by extending to deeper soil layers. Based on typical rooting depths found in agricultural crops and pastures and the capacity of deeper soil horizons to sequester relatively large amounts of soil organic C, there is evidence to suggest that measurements should extend to deeper layers (Fan et al., 2016; Lorenz and Lal, 2005; Viscarra Rossel et al., 2016b).
Conventionally, the measurement of soil organic C stocks involves soil sampling (see Sect. 3.2), followed by sample preparation and laboratory analysis. For the analytical determination of soil C concentration, sample preparation typically entails drying, crushing, grinding, sieving, sub-sampling, quantification of the sample's water content, and further fine grinding for dry-combustion analysis (Nelson and Sommers, 1996; Rayment and Lyons, 2011). Conventional measurements of soil bulk density typically involve using the volumetric ring method, where a pit is dug and a metal core of known volume is driven into the soil at the fixed depth. The bulk density is then determined by dividing the oven-dry soil mass of the sample by the volume of the core (Blake and Hartge, 1986). Alternatively, the clod method (Cunningham and Matelski, 1968; Hirmas and Furquim, 2006; Muller and Hamilton, 1992) has been used for soil with abundant rock fragments, where clods of soil are sampled and sealed (e.g. with paraffin) and the volume of the sample is determined by its displacement of water in a vessel. Gravel content is conventionally measured by breaking the soil cores into specific depth intervals, drying them in an oven, crushing the soil with a mortar and pestle, and then sieving it to separate the fine-earth (≤ 2 mm) fraction from the gravel.
Equation (1) can be used to quantify and report the change in soil organic C stocks at fixed depth intervals. However, this method can systematically overestimate or underestimate C stocks if bulk densities increase or decrease, respectively, from changes in land use or land management practices (e.g. changes in cultivation). Where bulk densities differ between management practices or across time periods, more accurate estimates of the C stock and its change can be derived using measures of cumulative or equivalent soil masses per unit area (Wendt and Hauser, 2013). Various studies have recognized the importance of this approach, which also reduces the effect of depth of sampling errors (Ellert et al., 2001; Gifford and Roderick, 2003; Lee et al., 2009; VandenBygaart and Angers, 2006; Wendt and Hauser, 2013). Both measurement-based methods under the Australian ERF (Australian Government, 2014, 2018) use an equivalent soil mass (ESM) approach to quantify soil organic C stock change.
3.2 Soil sampling and estimation
Before measuring the soil organic C stocks (Eq. 1), a sampling design must be derived to determine the sampling locations. Methods to select sampling locations include probability sampling and non-probability sampling, which result in two widely used sampling philosophies: design- and model-based sampling (Brus and DeGruijter, 1993; Papritz and Webster, 1995; de Gruijter et al., 2006). In design-based sampling, the randomness of an observation originates from the random selection of sampling sites, whereas in model-based sampling, randomness comes from a random term in the model of the spatial variation, which is added to the model because our knowledge of the spatial variation is imperfect. Probability sampling is, therefore, a requirement for design-based sampling but not for model-based.
Choosing which approach to use depends mostly on purpose (Brus and de Gruijter, 1997). For instance, if one needs estimates of the mean or total soil organic C stock and their accuracy over a given area, whose quality is not dependent on the correctness of modelling assumptions, then design-based sampling might be most suitable. If the aim is to produce a map of the soil organic C stock over the area, then model-based sampling will be preferable. However, because design-based sampling can also be used for mapping and model-based sampling for the estimation of means or total C stocks, the choice of which approach to use can be difficult. Viscarra Rossel et al. (2016b) demonstrated the use of probability sampling, which allowed design-based, model-assisted, and model-based estimation of the total soil organic C stock across 2837 ha of grazing land in Australia. Spectroscopic and active gamma attenuation sensors were used for estimating soil organic C stocks and their accuracy in the 0–10, 0–30, and 0–100 cm layers and for mapping the stocks in each layer across the study area. Although the design-based, model-assisted, and model-based estimates of the total soil organic C stocks were similar, the variances of the model-based estimates were shown to be smaller than those of the design-based methods. The authors noted that the advantage of the design-based and model-assisted methods, unlike the model-based approach, was that their estimates of the baseline soil organic C stocks and their variances did not rely on the assumptions of a model. Further, they noted that although the model-based approach produced the smallest variance of the predicted total soil organic C stocks, the results cannot be generalized to other sample sizes and types of sampling designs. We suggest that whatever the method used, careful consideration of the sampling design is needed for the estimation of the baseline soil organic C stocks and for monitoring. Further discussion on the advantages and disadvantages of the sampling approaches can be found in de Gruijter et al. (2006).
We reviewed the literature on proximal soil sensing (see Viscarra Rossel et al., 2011 for a definition), to find the sensors that can be used to determine the soil organic C stock, that is, sensors to measure organic C concentration, bulk density, and gravel content (Eq. 1). Table 2 summarizes the assessment of each sensor technology in terms of their rapidity, accuracy, cost, safety, readiness for field-deployment, and stage of research and development. Below we also evaluate and report their suitability for measuring soil organic C and for monitoring its change.
We did not consider mobile spectroscopic sensing (Christy, 2008) in this review. Although these systems can be used to produce maps of soil organic C concentrations, which could be used in the sampling design and for the estimation of the C stocks, they are insufficient for soil organic C accounting because they do not measure C stocks; they do not measure the same depth consistently, and measurements are often made only within the 0–20 cm layer, which is shallower than the recommended 0–30 cm minimum depth of measurement for soil C accounting.
Table 2Assessment of proximal sensing technologies regarding their readiness to underpin carbon accounting methodologies. The sensing methods are visible–near-infrared (vis–NIR) and mid-infrared (mid-IR) diffuse reflectance spectroscopy; laser-induced breakdown spectroscopy (LIBS); inelastic neutron scattering (INS); active gamma-ray attenuation (AGA); gamma- and X-ray computed tomography (CT). A “?” indicates “unknown” or “not sufficiently developed”; accuracy is relative to the conventional dry-combustion method for soil organic C concentration, volumetric ring method for bulk density, and manual processing for gravel content; cost: $ – AUD 0–40 000; $$ – AUD 40 000–100 000; $$$ – AUD 100 000+; active source refers to whether the source of energy used by the sensor is radioactive.
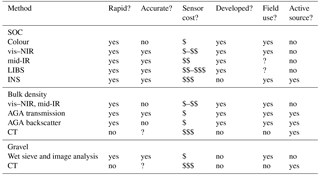
4.1 Sensing of soil organic C concentrations
4.1.1 Soil colour
Because organic C is known to affect soil colour, it is possible to use colour to estimate the organic C content of the soil (Ibañez Asensio et al., 2013; Liles et al., 2013; Viscarra Rossel et al., 2006a), for example, using digital cameras (Viscarra Rossel et al., 2008a) or mobile phone applications (Aitkenhead et al., 2016; Stiglitz et al., 2017). Although soil colour has been used to accurately predict soil organic matter content at regional and larger scales (Ibañez Asensio et al., 2013; Liles et al., 2013; Viscarra Rossel et al., 2008a), predictions are often inaccurate at field–farm scales or with soil that has inherently small C concentrations.
4.1.2 Soil visible, near-, and mid-infrared spectroscopy
Spectroscopic methods characterize soil organic C according to absorptions at specific wavelengths in the given spectral region. Visible and infrared spectroscopic techniques are highly sensitive to both the organic and inorganic components of soil, making their use in the agricultural and environmental sciences particularly relevant. Absorptions in the visible (vis: 400–700 nm) portion of the electromagnetic spectrum are due to electronic transitions and are useful for characterizing organic matter in soil as well as iron-oxide mineralogy (Sherman and Waite, 1985). Absorptions in the near infrared (NIR: 700–2500 nm) correspond to overtones and combinations of fundamental absorptions that occur in the mid-infrared region (mid-IR: 2500 and 25 000 nm) (Williams and Norris, 2001). As a consequence, absorptions in the NIR range are weaker and less distinctive compared to those in the mid-IR. It is useful to combine the vis and NIR ranges as each provides complementary information on soil. Instrument manufacturers have recognized this, and many offer spectrometers that measure the vis–NIR range.
Visible–NIR spectroscopy has been used successfully to predict soil organic C concentration, even under field conditions, but in the latter case using a method for correcting or removing the effects of soil water on the vis–NIR spectra (Ji et al., 2015; Minasny et al., 2011). Mid-IR can also accurately predict soil organic C concentrations. However, mid-IR spectroscopy is used mainly in the laboratory with measurements on oven- or air-dried and finely ground (typically 80–500 µm) soil samples (Le Guillou et al., 2015; Reeves, 2010; Reeves et al., 2012). There are strong water absorptions in the mid-IR, which tend to either mask or deform absorptions due to other soil constituents, thus degrading the calibrations and predictions of soil organic C with mid-IR spectra. There are published reviews on vis–NIR and mid-IR spectroscopy for predicting soil properties, including soil organic C concentrations (Bellon-Maurel and McBratney, 2011; Kuang et al., 2012; Soriano-Disla et al., 2014; Stenberg et al., 2010; Viscarra Rossel et al., 2006b, 2016a). We direct the reader to those reviews for further details.
To predict soil organic C, the spectroscopic techniques described above require the development of an empirical model (or calibration) that relates the spectra to corresponding soil data analysed with a reference analytical method such as dry-combustion analysis. This data set, which holds the spectra, soil analytical data, and metadata is referred to as a spectral library. To be useful for site-specific predictions of soil organic C, the spectral library should contain data that represent the local variability of soil organic C concentration. In Sect. 5.2 below, we review the methods that can be used to derive spectroscopic calibrations for predictions of soil organic C concentrations.
4.1.3 Laser-induced breakdown spectroscopy
Laser-induced breakdown spectroscopy (LIBS) uses atomic emission spectroscopy. A focused laser pulse heats the surface of the soil sample to break the chemical bonds and vaporise it, generating a high-temperature plasma on the surface of the sample. The resulting emission spectrum is then analysed using a spectrometer covering a spectral range from 190 to 1000 nm. The different LIBS peaks from the analysed samples can be used to identify the elemental composition of the soil. Information on peak intensities can then be used to quantify the concentration of elements in the sample. Cremers and Radziemski (2006) and Senesi and Senesi (2016) provide detailed descriptions of the method.
Reports that use LIBS for measuring soil organic C mostly use large benchtop instruments with prepared samples and calibrations to predict soil organic C from the measured elemental C (Bel'kov et al., 2008; Cremers et al., 2001; Ebinger et al., 2003; Knadel et al., 2017). These studies have reported good correlations between LIBS measurements and those from dry combustion, particularly for soil with similar morphology (Cremers et al., 2001; Ebinger et al., 2003). They also reported that LIBS measurements are rapid (less than a minute per sample). LIBS can provide rapid and accurate soil elemental analysis on prepared soil samples, and evidently, LIBS spectra can be calibrated to estimate soil organic C.
Currently, the primary constraints of LIBS for measuring soil organic C are sample preparation, sample representativeness because only a tiny volume of soil is ablated (Izaurralde et al., 2013), and our limited understanding of the accuracy of its measurements on soil that is wet under field conditions. We are aware of only one study that reports the use of LIBS for measuring the organic C content of intact, field-moist soil core samples in the laboratory (Bricklemyer et al., 2011). In that study, Bricklemyer et al. (2011) evaluated the accuracy of a field-scale LIBS calibrations for estimating total, inorganic, and organic C concentrations. The authors showed that LIBS spectra recorded from intact soil cores could be calibrated to quite accurately estimate total C and inorganic C concentrations. However, estimates of soil organic C were poor (r2 = 0.22). The authors suggested that the poor predictability might be due to the low variance of organic C in their soil samples (Bricklemyer et al., 2011). Another factor that may have contributed to the poor predictability may be the narrow spectral range, which did not capture emissions from several other elements associated with organic C (Senesi and Senesi, 2016).
Although there are some commercially available portable LIBS systems (Harmon et al., 2005), there are few reports on their use for measuring soil organic C. Da Silva et al. (2008) used a portable LIBS system to measure a small number of pre-processed tropical soil samples in the laboratory. Izaurralde et al. (2013) used an “SUV-portable” LIBS system for field measurements of soil organic C, but significant sample processing was needed before measurements could be made, including breaking up soil cores and pelleting sub-samples in a hydraulic press. The authors speculated that there is potential for using LIBS to measure intact soil cores under field conditions (wet), albeit less accurately. More research and development is needed to assess the potential for LIBS to accurately estimate soil organic C concentrations in soil that is under field conditions.
4.1.4 Inelastic neutron scattering
Inelastic neutron scattering (INS) involves spectroscopy of gamma rays induced by fast and thermal neutrons interacting with the nuclei of the elements in soil. Fast neutrons, generated by a neutron generator, penetrate the soil and stimulate gamma rays that are then detected by an array of scintillation detectors such as sodium iodide (NaI) detectors. Peak areas in the measured spectra are proportional to the elemental composition of the soil, and peak intensities (counts) together with established calibrations can be used to determine soil organic C in units of g C m−2 (Wielopolski et al., 2008).
The suggested benefits of INS include the ability to interrogate large volumes of soil over a relatively large footprint and the ability to measure to a depth of approximately 30–50 cm (Chatterjee et al., 2009). Thus, there is good potential for using it to non-invasively measure soil organic C (volumetrically) to a depth of around 30 cm. There is also no sample preparation required. Wielopolski et al. (2011) reported the feasibility of an INS instrument for measuring soil organic C. The sensor used high-energy neutrons and photons to sample soil volumes up to about 0.3 m3 and from approximately 20 to 30 cm deep, with a 150 cm diameter footprint. Although this technology appears useful, it is not yet sufficiently developed, the equipment is expensive, and there are concerns around the safe use of fast neutron generators in farms (Izaurralde et al., 2013).
4.2 Sensing of soil bulk density
4.2.1 Active gamma-ray attenuation
Active gamma-ray attenuation (AGA) measures the attenuation of the radiation by the soil, as defined by Beer–Lambert's Law, and provides a direct measure of the soil density. Because the mass attenuation coefficient of soil is a function of both photon energy and its elemental composition, attenuation is affected by texture and mineralogy. The measurement of bulk density by AGA can be made using either the scattering method or the transmission method. The former is applied to mostly surface determinations, mainly using gamma/neutron surface gauges, while the latter is used for measurements at depth, which can be made in the laboratory or the field (Pires et al., 2009).
AGA with a gamma (or neutron) surface gauge and a source of radiation that is lowered into the soil to the effective measurement depth can be used to measure soil density. The backscattered gamma radiation that originates from the source loses some of its energy on the way back to the scintillation detector at the surface, and the energy of the detected radiation is proportional to the density of the soil. The technique requires considerable soil preparation and correction for soil water to derive soil bulk density. Soil surface preparation requires that there are no gaps between the soil and the sensor, and, for measurements at depth, a pit needs to be dug to the effective measurement depth into which the active gamma source is lowered. Reports on the accuracy of these measurements are variable (Holmes et al., 2011; Timm et al., 2005) and are possibly due to problems with uneven soil surfaces and soil preparation issues. Relationships between bulk density measured with a neutron density meter and those on paired soil samples made with the conventional ring method were not strong, and they were variable (R2 = 0.14–0.47, N = 75; Holmes et al., 2011). Others needed new calibrations for different soil types or bulk densities < 1.4 g cm−3 (C'assaro et al., 2000; Rousseva et al., 1988). Other studies have shown that bulk density measured with a neutron–gamma surface gauge tended to be less dense than the conventional ring method, although differences were not statistically significant (Bertuzzi et al., 1987; Rawitz et al., 1982; Timm et al., 2005).
AGA using measurements of transmission can be used to measure soil density. In this case, the measurements are made axially through a soil core and the attenuation of gamma radiation passing through it to the scintillation detector is proportional to the density of the soil. Pires et al. (2009) and Lobsey and Viscarra Rossel (2016) provide descriptions of the measurement principles. The method requires sampling of intact soil cores and when measurements are made on soil under field condition, corrections for water, θ, are needed. No other sample preparation is required.
The bulk density of the soil cores, ρb, can be derived with (Lobsey and Viscarra Rossel, 2016):
where I is the incident radiation at the detector, I0 is the un-attenuated radiation emitted from the source, x is the sample thickness in cm, μs is the mass attenuation coefficient of dry soil in cm2 g−1, μw is the mass attenuation coefficient of soil water at 0.662 MeV, ρw is the density of water (taken as 1 g cm−3), and θ is the volumetric water content of the soil in cm−3 cm−3.
Good agreement between measures of bulk density with an AGA transmission sensor and the conventional volumetric ring method has been found for dry samples in the laboratory (Pires et al., 2009). More recently, Lobsey and Viscarra Rossel (2016) showed that this method with vis–NIR corrections for water could accurately and rapidly (on average 35 s per measurement) measure, ex situ, the bulk density of soil cores sampled (wet) under field condition. The method facilitates the analysis of soil bulk density at fine depth resolution enabling the characterization of the spatial variability of soil bulk density in lateral and vertical directions. Lobsey and Viscarra Rossel (2016) report that the accuracy of measurements was similar to that obtained using the conventional single-ring method on the same samples (RMSE = 0.06 g cm−3; R2 = 0.90; N = 32). Further, the authors show that the method can be used to determine organic C stocks on a fixed-depth or equivalent soil mass basis (Lobsey and Viscarra Rossel, 2016).
4.2.2 Computed tomography
Computed tomography (CT) was introduced in soil science several decades ago (Petrovic et al., 1982). Since then, it has been used to assess porosity and pore size distribution (inversely related to bulk density), tortuosity, soil structure, and compaction (Lopes et al., 1999; Pires et al., 2010). CT is based on the principle that electromagnetic radiation (commonly X- or gamma rays), is attenuated by matter. Similar to the AGA described above, attenuation follows the Beer–Lambert Law. CT is used to convert the attenuation of the radiation by matter into CT numbers called tomographic units (TUs), and the soil mass attenuation coefficient is used to derive soil density. The techniques can produce cross-sectional images to create a three-dimensional model, and hence they have good potential for measuring soil bulk density (and gravel content; see Sect. 4.3.1 below) of intact soil core samples.
Only a few studies have demonstrated that gamma-ray CT can be used to measure soil bulk density (Pedrotti et al., 2005; Timm et al., 2005). Timm et al. (2005) compared measurements of bulk density with gamma-ray CT to several other methods, including the conventional volumetric single-ring method, and found the CT technique to be more accurate. In an evaluation of the potential for X-ray microtomography for measuring the bulk density of soil with different textures and at different depths, Segnini et al. (2014) found only moderate linear agreement with the conventional volumetric ring method (R2 = 0.58, N = 12). They concluded that factors such as the “beam hardening” effect (see Cnudde and Boone, 2013) and the polychromatic nature of X-ray microtomography make it difficult to measure soil bulk density directly. However, more research and evaluation is needed. An advantage of the CT methods is that they can provide a detailed analysis of soil bulk density profiles at a fine spatial resolution. Cnudde and Boone (2013) provide a review of the applications and limitation of X-ray CT.
4.2.3 Spectroscopic and pedotransfer functions
The bulk density of soil is a measure of the amount of pore space in a volume of soil. Thus, spectroscopy, being a surface measurement, cannot physically measure density, particularly if the soil has been ground and sieved. Nonetheless, it is often suggested that predictions of bulk density using vis–NIR or mid-IR spectra are possible. The reason is that under certain conditions, these spectroscopic models rely on second- or higher-order correlations to other soil constituents that are spectroscopically active (e.g. minerals, organic matter, water). However, because the predictions are “indirect”, they can be biased. Moreira et al. (2009) found that using vis–NIR spectroscopy on dry soils to predict soil bulk density produced inaccurate results and concluded that further research was needed to assess the limits and specificity of the method. A more recent study by Roudier et al. (2015), using vis–NIR on wet intact cores, found that predictions of bulk density were relatively accurate (in soil containing no gravel), but calibration was at a very local scale and thus for very specific conditions. Pedotransfer functions (PTFs) are commonly used to estimate bulk density (Tranter et al., 2007). However, they are often biased and imprecise and therefore unsuitable for the determination of soil organic C stocks, even when developed with soil from the same study area (Don et al., 2007). A further disadvantage of using PTFs is that they use other soil properties, which need to be measured, as input variables.
4.3 Sensing of gravel
Gravels are defined as coarse fragments with particles that are coarser than 2 mm (McKenzie et al., 2002). The presence of gravel has a significant effect on the mechanical and hydraulic properties of soil (Brakensiek and Rawls, 1994; Sauer and Logsdon, 2002). If gravel is present but not accounted for, it could bias the measurements of soil organic C stocks (Lobsey and Viscarra Rossel, 2016; Poeplau et al., 2017). For example, the presence of abundant coarse fragments (> 20 %) adversely affected the measurement of soil bulk density by both conventional and AGA using backscatter methods (Holmes et al., 2011). Sensing of gravel is difficult, and so it is typically measured manually by drying, crushing, sieving, and weighing of the soil and gravel. This method is time-consuming.
4.3.1 Wet sieving and image analysis
Viscarra Rossel and Lobsey (2017) developed a wet-sieving system combined with image analysis to more efficiently measure the gravel content of soil core samples in the field. The system enables rapid wet sieving of the core samples in 10 cm increments. The system is modular and can accommodate soil cores of various lengths. The authors tested the system using four soil types with varying textures and gravel contents. They showed that for a 1 m soil core, gravel could be separated from the soil, at 10 cm intervals, in 10–20 min, which is considerably faster (by a factor of more than 10) than the conventional method. By imaging the resulting gravel and measuring the pixel area or pixel volume occupied by the gravel in the images, they could accurately estimate the gravel content of the different soil types compared with manual weighing (R2 = 0.79–0.90, average R2 = 0.85 over the four soil types; Viscarra Rossel and Lobsey, 2017). The authors suggested that the method showed good promise for use in a soil C stock measuring system and that further testing and improvements of the wet sieving might include using a dispersing agent (e.g. (NaPO3)6). A further advantage of the method is that as well as gravel, un-decomposed plant materials and roots are also separated (Viscarra Rossel and Lobsey, 2017).
4.3.2 Computed tomography
There is good potential for the development of CT methods to measure gravel (and bulk density). However, there is little research on the topic. Fouinat et al. (2017) tested a novel X-ray CT method to analyse distinct deposits in lake sediment cores. The analysis highlighted the presence of denser > 2 mm mineralogical particles (i.e. gravel) in the silty sedimentary matrix. When compared to conventional manual measurements that involved sieving and water displacement to measure volume, they found that CT measurements overestimated the volume of the gravel by 11.6 %. The authors suggested that the overestimation might be due to pixel resolution issues. Nonetheless, the authors obtained a strong positive correlation (ρ = 0.81) between the CT measurements and the more conventional method. More research and development is needed, and the discussion above for sensing bulk density also applies here for gravel.
The development of CT for quantifying both gravel (and bulk density) appears promising. Portable CT scanners exist for medical and other applications, including soil, but further research and development or significant modification of existing systems are needed to measure bulk density and gravel content for C accounting. The system would need to have (1) the ability to rotate the soil core around fixed sensors or rotate the sensors around the core; (2) short measurement times to produce images of appropriate resolution; (3) adequate emission energies and appropriate shielding; (4) specialized software for the reconstruction of images and measurement of bulk density and gravel; and (5) appropriate size and weight to allow routine field deployment. Of course, a vis–NIR sensor for measuring soil organic C concentration would need to be used with it. In the meantime, however, the separation of gravel by rapid wet sieving and quantification by automated weighing or by image analysis might be an efficient interim.
4.4 Evaluation of sensing for soil organic C accounting
Based on our review and assessment of the available sensor technologies above, currently, the most suitable proximal sensing techniques for measuring soil organic C and for monitoring its change are vis–NIR and mid-IR spectroscopy for estimating soil organic C concentration and AGA for measuring bulk density. There are no practical or efficient sensors available for measuring gravel. Presently, a possible best option might be wet sieving to separate the gravel fraction and quantification by weighing or image analysis (Viscarra Rossel and Lobsey, 2017). Table 3 provides a summary of the benefits and limitations of each of these technologies, and Table 4 assesses their cost and accuracy.
Table 3Benefits and limitations of sensing technologies for soil C accounting. vis–NIR – visible and near infrared diffuse reflectance spectroscopy; mid-IR – mid-infrared spectroscopy; AGA – active gamma-ray attenuation; ESM – equivalent soil mass; SOP – standard operating procedure.
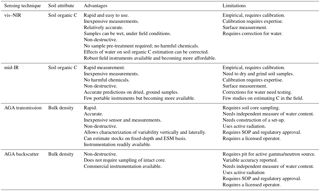
Table 4Assessment of the accuracy and cost of sensing technologies for soil C accounting. The methods are visible–near infrared (vis–NIR) and mid-infrared (mid-IR) diffuse reflectance spectroscopy; active gamma-ray attenuation (AGA) and wet sieving and image analysis. The accuracy at local scale (i) for soil organic C concentrations is based on comparison with dry-combustion analysis on the same samples and represented by median values for dry soil samples (vis–NIR and mid-IR) and wet samples (vis–NIR only); (ii) for bulk density is based on a comparison with the volumetric ring method and represented by median values for wet soil corrected for water with vis–NIR measurements on the same samples (transmission) or for wet soil corrected for gravimetric water content on paired samples (backscatter); and (iii) for gravel content is based on a comparison of image analysis with weighing on the same wet-sieved samples and represented by median values. The statistics reported are the root mean square error of validation (RMSEv), the coefficient of determination (), and the ratio of performance to deviation (RPDv). N are the number of local sites at which accuracy was assessed.
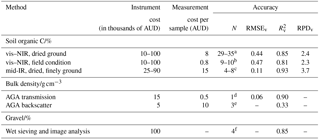
a Sourced from Sankey et al. (2008b), Roudier et al. (2017), Chodak et al. (2007), Conforti et al. (2017), Fontan et al. (2010), Martin et al. (2002), McCarty and Reeves (2006), Nduwamungu et al. (2009), Peng et al. (2013, 2014), Araujo et al. (2015), Rodionov et al. (2014), Sarkhot et al. (2011), Shi et al. (2014), Udelhoven et al. (2003), Vagen et al. (2006), Vohland and Emmerling (2011), and Yang et al. (2012). b Sourced from Viscarra Rossel et al. (2017), Roudier et al. (2015), Wetterlind and Stenberg (2010), and Rodionov et al. (2014). c Sourced from Viscarra Rossel et al. (2006b), Araujo et al. (2015), McCarty and Reeves (2006), Bornemann et al. (2010), Peng et al. (2014), and Yang et al. (2012). d Sourced from Lobsey and Viscarra Rossel (2016). e Sourced from Holmes et al. (2011). f Sourced from Viscarra Rossel and Lobsey (2017).
The cost of spectrometers can vary widely (Table 4). Portable vis–NIR spectrometers (350–2500 nm) can be purchased from different manufacturers from approximately less than AUD 10 000 to more than AUD 100 000 (Table 4), although their cost is continually decreasing as technologies develop. Smaller, cheaper vis–NIR spectrometers that use micro-electromechanical systems (MEMSs) technologies are emerging and are less expensive, but not many have been thoroughly tested. Prices for mid-IR portable spectrometers (2500–20 000 nm) are approximately AUD 50 000–70 000, and for mid-IR benchtop spectrometers, they are approximately AUD 25 000–90 000, depending on the size, type of detector, sensitivity, and amount of automation. The cost of spectroscopic measurements of soil organic C concentration in the laboratory is larger than measurements under field conditions because of the need for sieving, drying, and grinding. mid-IR measurements are expensive because samples need to be finely ground. Both vis–NIR and mid-IR techniques can accurately predict the soil organic C content of dry soils (Table 4). The accuracy of vis–NIR predictions on wet soil, that is under field conditions, is generally less than that for dry soil, although the difference can be relatively small (Table 4).
AGA sensors for measuring bulk density are also quite readily available (Table 4). Measurement costs are significantly smaller for AGA using transmission than for AGA using backscatter because of the additional soil preparation required for the latter. There are few reports on the use of AGA for the measurement of bulk density, but they report good accuracy for AGA using transmission and variable accuracy for AGA using backscatter. There is no information on the cost and accuracy of wet sieving and image analysis for quantifying gravel (Table 4). Viscarra Rossel and Lobsey (2017) suggested that a system could be easily developed and that measurement costs would be small.
4.5 Integrated multi-sensor systems for soil organic C accounting
We need an integrated multi-sensor approach for measuring and monitoring soil organic C stocks because simultaneous measurements of soil organic C concentration, bulk density, and gravel are needed. There are two currently available, field-deployable, proximal multi-sensor systems to measure soil organic C stocks. One involves inserting the sensors into the soil profile and making measurements in situ, while the other requires sampling undisturbed soil cores and measuring the soil ex situ.
Veris® Technologies produces commercial sensors for precision agriculture (http://www.veristech.com, last access: 6 December 2017), including several field-deployable systems which can measure electrical conductivity, pH, and penetration resistance and also record the vis–NIR spectra of soil. The system that is particularly relevant to soil organic C accounting is the P4000, which uses a hydraulic probe system to insert four sensors into the soil to characterize the profile. The sensors are a vis–NIR spectrometer (350–2200 nm), an electrical conductivity (EC) sensor, and an insertion force sensor. The system does not measure bulk density. Using insertion force as a surrogate for bulk density might be possible but would be prone to errors. Wetterlind et al. (2015) tested the accuracy of predictions of soil organic matter (SOM) using the P4000 system and evaluated whether the predictions were improved when the sensors were combined. They found that the accuracy of predictions of soil organic matter content with the vis–NIR alone was good, but the inclusion of insertion force only improved prediction accuracy by about 10 %. They concluded that these small improvements did not provide strong support for combining vis–NIR sensor measurements with measurements of insertion force. However, there was no testing of bulk density.
The Soil Condition Analysis System (SCANS) (Viscarra Rossel et al., 2017) uses a combination of proximal sensing technologies, smart engineering, and data analytics to characterize soil laterally across the landscape and vertically down the profile. The SCANS has an automated soil core sensing system, which can be used in the laboratory or the field. The system has a vis–NIR (350–2500 nm) spectrometer, an AGA densitometer, and digital cameras that measure intact soil core samples that are either (wet) under field condition or dry, at user-defined intervals over the length of 1.2 m soil cores. The system can measure soil organic C content and composition (particulate, humus, and resistant C), bulk density, clay content, cation exchange capacity, volumetric water content, available water capacity, pH, iron, and clay mineralogy (Viscarra Rossel et al., 2017). Each measurement with the sensors takes approximately 35 s so that measuring a 1 m core at 2 cm intervals (i.e. 50 measurements along the core) takes about 30 min. Viscarra Rossel et al. (2016b) showed that the sensing system could be used to accurately baseline soil C stocks for accounting purposes, and Viscarra Rossel and Brus (2018) assessed its cost-efficiency and reliability for soil C accounting. They found that compared to more conventional methods that use composite sampling and laboratory analysis, sensing with the SCANS is more cost-efficient in that it provides a good balance between accuracy and cost.
The rationale for using sensing in a method for soil organic C accounting is that although sensing may not be as precise per individual measurement compared to laboratory analysis, sensing is more cost-efficient; that is, sensing provides a balance between accuracy and cost. Because sensing is cheaper, simpler, and more practical to use, many more measurements can be made across space (laterally and vertically) and time, so that as an ensemble, the data are more informative. Sensing can also be non-destructive, allowing the soil samples to be stored in archives for future measurement should auditing and verification be required. The archived soil samples can then also ensure that there is consistent temporal data for use in dynamic models or for the testing of new technologies and approaches as they become available. Below we describe considerations needed for developing soil organic C accounting methodologies with proximal sensing.
5.1 Development of spectral libraries
The measurement of soil organic C using spectroscopy (e.g. vis–NIR, mid-IR) requires the calibration of the spectra to soil organic C content using multivariate statistics or machine-learning algorithms. The calibrations can be derived using existing large spectral libraries (ESLs) (Shepherd and Walsh, 2002; Stevens et al., 2013; Viscarra Rossel and Webster, 2012) or using new site-specific libraries developed with local soil samples (LSLs). Using an ESL to predict soil organic C incurs no immediate cost, but it is likely that the predictions at the local site (farm or field scales) will be biased (Clairotte et al., 2016; Guerrero et al., 2014b). Using an LSL will produce more accurate (unbiased) predictions but will incur a cost because soil needs to be analysed in the laboratory to derive the local model.
Significant investment has been made in developing large regional, country, and global spectral libraries (Brown et al., 2006; Shepherd and Walsh, 2002; Viscarra Rossel et al., 2016a), and there will be value in using these for developing site-specific calibrations. These ESLs could reduce the need for site-specific data. Various approaches have been proposed to make better use of ESLs for local predictions of soil properties. They are based on either constraining the ESL with spectral or soil sample similarities or augmenting the ESL with site-specific samples.
Memory-based learning (MBL) methods aim to constrain the ESL with spectral information and derive calibrations for each unknown sample on a case-by-case basis. By selecting a subset of the ESL to predict each unknown sample, these methods effectively derive site-specific (i.e. local) calibrations. Methods include the LOCAL (Shenk et al., 1997) and locally weighted regression (LWR) (Naes et al., 1990) algorithms and their variants. Essentially, the methods select calibration samples from the ESL with a distance metric (e.g. Mahalanobis distance) in the multivariate space between the calibration and the unknown samples. In LWR weighting the calibration samples are also weighted according to their spectral dissimilarity (distance) to the unknown samples.
Ramirez-Lopez et al. (2013) proposed spectrum-based learning (SBL), which is a type of MBL. The spectrum-based learner selects nearest neighbours from an ESL using distance metrics calculated in the principal component space and optimizing the number of components used to identify the nearest neighbours in the selection. Spectroscopic modelling is then carried out with both the selected neighbours and the matrix of distances to the unknown samples as the training data set.
The ESL can also be constrained with other information such as soil order, type, texture, and parent material (Sankey et al., 2008a; Vasques et al., 2010). Shi et al. (2015) proposed the use of both spectral similarities and geographically constrained local calibrations to predict soil organic C content. They reported improvements in the accuracy of predictions when the ESL was restricted to the geographic region from which the unknown samples originated. Viscarra Rossel and Webster (2012) developed general ESL calibrations for Australian soil using the machine learning algorithm Cubist. The authors showed that the algorithm makes inherently local predictions because Cubist partitions the spectra into local subsets that are each modelled separately.
Two techniques use the augmenting approach. They are “spiking”, which uses several local spectra to augment the calibration made with an ESL (Guerrero et al., 2010; Sankey et al., 2008b; Viscarra Rossel et al., 2009), and spiking with extra-weighting (Guerrero et al., 2014a), which uses multiple copies of the local samples to improve their leverage in the calibrations. Guerrero et al. (2014a) showed that the latter approach improved on spiking and suggested it might be more appropriate with larger spectral libraries.
Lobsey et al. (2017) developed a new approach, which they call rs-local, which makes the best use of ESLs and minimizes the number of site-specific, local samples for deriving calibrations. The method is data-driven and makes no assumptions on spectral or sample similarities. Using data from farms in Australia and New Zealand, they showed that by combining 12–20 local samples with a well-selected set of samples from an ESL, the robustness and accuracy of the predictions was improved compared to predictions made using a “general” calibration and other methods tested. The authors suggested that rs-local can reduce analytical cost and improve the financial viability of soil spectroscopy.
5.2 Spectroscopic modelling: training, validation, and prediction
As described above, to measure and monitor soil organic C with spectra, a local spectroscopic model needs to be developed to ensure that the estimates of organic C are unbiased. Therefore, once the soil in a study area has been sampled, according to an appropriate soil sampling design (see Sect. 3.2), the spectra of the sampling units in the sample should be recorded using standardized protocols and guidelines, such as those described by the Global Spectral Library in Viscarra Rossel et al. (2016a). Following a spectral outlier analysis to identify erroneous spectra (due to rocks, roots, and other non-soil materials), the spectra of the sample, which characterizes the variability in the study area, are used to guide the selection of data for the spectroscopic modelling and prediction, i.e. the training, validation, and prediction sets.
A method that ensures that the training spectra adequately represent the sample must be used to select the training set e.g. the Kennard–Stone (Kennard and Stone, 1969) or Duplex (Snee, 1977) algorithms. The validation set should be selected by random sampling to ensure an unbiased assessment of the spectroscopic model predictions. How many spectra to use for training and validation depends on the available budget because the selected sampling units will need to be analysed with conventional laboratory methods and on the heterogeneity of the sample. Spectroscopic models for the prediction of soil organic C that are developed and validated with too few data can lead to unstable and erroneous results (Reeves et al., 2012). Once the total number of sampling units are selected for the spectroscopic modelling, a general rule of thumb is to use two-thirds for training and the remaining third for validation, although this is not a hard rule and the choice might depend on the total number of sampling units that are selected. The prediction set is made up of the data that remain in the sample after the training and validation sets have been selected.
Once the spectra for the modelling have been selected, soil aliquots of the respective sampling units need to be prepared for the analysis of soil organic C concentrations in the laboratory by dry combustion analysis (e.g. LECO – Laboratory Equipment Corporation). It is important to note that the inaccuracy and imprecision of analytical results are directly related to the sampling, handling, and analytical procedure (Viscarra Rossel and McBratney, 1998). Therefore, soil sample preparation (drying, crushing, grinding, sub-sampling) and analytical measurements should be made with certified methods and in an accredited laboratory that conducts regular technical and inter-laboratory proficiency programs. For example in Australia accreditation is though the National Association of Testing Authorities (NATA) and the Australian Soil and Plant Analysis Council (ASPAC). We recommend that an independent assessment of the analytical accuracy is performed by including a small but representative proportion of “blind” duplicates (e.g. 15 %) in the analysis. If the blind duplicate samples exceed a predetermined threshold value (e.g. 0.05 % soil organic C), then the samples should be re-analysed by the laboratory. As with any modelling, the dictum when developing spectroscopic calibrations is “garbage in = garbage out” and conversely “quality in = quality out” (Viscarra Rossel et al., 2008b).
The spectra and analytical data in the training set should then be analysed and if necessary, transformed, pre-processed, and pre-treated. For example, if the algorithm for the modelling assumes that the response variable is normally distributed, then the analytical data will need to be checked and if necessary transformed (e.g. with logarithmic transforms) to approximate a normal distribution. Similarly, the spectra may need a transformation to apparent absorbance; it may need smoothing and baselining (e.g. using a Savitzky–Golay filter with a first derivative; Savitzky and Golay, 1964). The spectra may also need to be mean-centred, and if recorded at field conditions, it may need corrections to remove the effects of water on the spectra (e.g. with either the external parameter orthogonalization – EPO, Minasny et al., 2011, or direct standardization – DS, Ji et al., 2015). See Roudier et al. (2017) for a comparison of EPO and DS. It is important to note that whatever transformations, pre-processing, and pre-treatments are applied to the training set, they must also be applied to the validation and prediction sets.
Before embarking on the modelling, it is sensible to check for outliers and influential data in the training set objectively. This can be done by calculating Studentized residuals (Cook and Weisberg, 1982), to check for observations with unusually large residuals or data that deviate significantly from their mean, i.e. those with high leverage (Martens and Naes, 1989). If outliers are detected, then further checks for data entry and other errors should be made. One should not remove data unless there is reasonable evidence to suggest that the data are in error.
Spectroscopic models should be developed by cross-validation to obtain optimal parameterization of the models and to minimize or prevent problems with under- or over-fitting. Once a model is developed, model diagnostics should be performed to interpret the model and also to check that the statistical assumptions of the particular algorithm being used are not violated. For example, this could simply be done by calculating the residuals of the data in the training set and plotting these against the estimated soil organic C concentrations. This plot can help to diagnose dependence of the predicted value, non-constant (or heteroscedastic) variances, and non-linear trends that indicate the need for data transformations or alternate curvilinear modelling methods (see for example, Martens and Naes, 1989).
If all assumptions about the model are correct and the model has a good diagnosis, then the optimized model should be validated with the independent validation set, which was selected at random and which was not used in the training process. The type of algorithm used (e.g. partial least squares regression (PLSR), support vector machines (SVMs), regression trees) is not critical as long as the optimization and validation are done well. Modelling uncertainties could be derived with Monte Carlo (Viscarra Rossel, 2007) or Bayesian methods.
The predictions on the validation set should be assessed with statistics that completely describe the errors in the same units as the analyte (i.e. soil organic C content). For this, we recommend the use of the RMSE, which measures the inaccuracy of the model predictions, the mean error (ME), which measures their bias, and the standard deviation of the error (SDE), which measures their imprecision. Inaccuracy may be defined as combining both bias and imprecision, so that RMSE2 = ME2 + SDE2 (Viscarra Rossel and Webster, 2012). Other indices that are commonly reported are the coefficient of determination (R2), the ratio of performance to deviation (RPD) (Williams and Norris, 2001), or the ratio of performance to interquartile range (RPIQ) (Bellon-Maurel et al., 2010) and the concordance correlation coefficient (Lin, 1989). We do not recommend the use of the R2 alone because it does not account for bias in the model predictions or the RPD or RPIQ alone because their categories are subjective and variable (Reeves and Smith, 2009).
If the independent model validation statistics are not too dissimilar to those reported in the literature for soil organic C predictions at the field and farm scales, e.g. for vis–NIR spectroscopy 0.1–1.0 % organic C, with a median value of 0.3 % organic C (Viscarra Rossel et al., 2016a), we recommend that additional sampling units be selected to augment and potentially extend the range of the training set. These values should serve only as guidelines, and if the validation statistics fall outside of these ranges after the augmentation of the training set, then it might not be sensible to proceed with spectroscopy for the estimation of organic C.
The optimized and validated spectroscopic model may then be applied to all of the spectra: the training, validation, and prediction sets, to estimate consistently the soil organic C concentration of the entire sample set and their uncertainty. If the model was developed on a transformed organic C scale (e.g. square root or logarithmic), then the estimates need to be back-transformed to the original units.
Figure 1 summarizes the procedures for the spectroscopic measurement, modelling, and prediction of soil organic C.
5.3 Standards for auditing and verification
Standardization of the sensing methods and their procedural guidelines are needed if sensing is to underpin methodologies that help to account for soil organic C stock change. This is particularly important for international initiatives like “4 pour 1000”, which aim to demonstrate that soil can play an important role in mitigating climate change. Standards and guidelines are also essential in schemes that use financial incentives for landholders to adopt C sequestration practices (see Table 1). In this case, standards will help to ensure that only authentic abatement is credited. In the Australian ERF, methods need to comply with offsets integrity standards, which require that abatement is additional, eligible, measurable, verifiable, evidence-based, statistically defensible, supported by relevant peer-reviewed scientific results, permanent, with no leakage, and conservative (see Sect. 2).
Bispo et al. (2017) reported that some standards exist for the analysis of soil properties, including organic C, but suggested that new standards are needed for the measurement of soil C stocks and the verification of C change. In Table 5, we propose a list of data and information that need to be reported when developing a sensing methodology for soil C accounting. These include, for instance, the type of sensor used, the requirements for calibration, the number of reference analyses to use, the requirements for validation, the statistics to report, and the information that must be recorded for auditing and verification. Of course, the list in Table 5, is additional to data on the project area, the sampling design used, the soil sampling method, the method for estimation, and the preparation of the samples for analysis.
Currently, the most suitable proximal sensing techniques for soil C (stocks) accounting are vis–NIR spectroscopy for estimating organic C concentration and active gamma attenuation for measuring bulk density. There are no practical or well-developed sensors for measuring gravel content in the soil. CT appears promising for measuring both bulk density and gravel, but there are no systems available for this specific purpose, and so the approach requires significant research and development. A useful interim for measuring gravel might be its separation by rapid wet sieving and quantification by automated weighing or image analysis.
The use of mid-IR spectrometers for measuring organic C under field condition needs further research and development. Laboratory measurements of soil with mid-IR are possible, but additional transport, sample preparation by drying, and fine grinding will incur more labour costs. Although other technologies, such as LIBS and INS have some advantages, they also have significant disadvantages and are currently not sufficiently developed for making cost-efficient measurements.
Sensing of soil organic C with spectrometers requires a multivariate calibration. There has been significant investment made to develop large regional, country, and global spectral libraries, and we believe that there will be value in using them to improve the accuracy and cost-efficiency of soil spectroscopy. Statistical data-driven methods are being developed that use such libraries and reduce the need for local samples for deriving site-specific calibrations. Nevertheless, there is a need to develop sensors that are more direct (i.e. less reliant on empirical calibrations), accurate, safe, and inexpensive for measuring soil organic C.
Cost-efficient organic C accounting requires that the individual sensing techniques, above, be combined in a field-deployable, integrated multi-sensor data-analytics system, to derive estimates of the C stocks in soil profiles from at least the top 30 cm, but preferably deeper. Such systems are currently being developed and are showing that sensing with vis–NIR and active gamma attenuation sensors can provide accurate, rapid, and cost-efficient estimates of C stocks on either a fixed-depth or an equivalent soil mass basis.
Sensing can be used to underpin soil C accounting methodologies and to evaluate land use and soil management practices that aim to increase soil organic C stocks, improve soil health, increase agricultural production, and mitigate GHG emissions. But to ensure proficient measurement and accurate reporting and verification, the sensing methods should be standardized, supported by peer-reviewed science, and covered by robust procedural guidelines. This is particularly important in schemes that use financial incentives for landholders to adopt management practices to sequester soil organic C.
The new legislated soil C accounting method under the Australian Emissions Reduction Fund allows practitioners to use sensors for C accounting. The method is the first in the world to do so, and it might provide a template for other countries to follow.
No data sets were used in this article.
RAVR conceived the work. JRE, and RAVR contributed to the writing and RAVR edited the final draft.
The authors declare that they have no conflict of interest.
This article is part of the special issue “Regional perspectives and challenges of soil organic carbon management and monitoring – a special issue from the Global Symposium on Soil Organic Carbon 2017”. It is a result of the Global Symposium on Soil Organic Carbon, Rome, Italy, 21–23 March 2017.
We thank the Australian Government Department of the Environment and Energy,
who procured work that led to the development of the review. We are grateful
to the CSIRO, the Department of Agriculture and Water Resources, and the Grains
Research and Development Corporation, who funded projects that led to the
development of some of the technologies and ideas presented in this paper.
We also thank Keryn Paul for commenting on our manuscript.
Edited by: Peter Finke
Reviewed by: two anonymous referees
Aitkenhead, M., Donnelly, D., Coull, M., and Gwatkin, R.: Estimating Soil Properties with a Mobile Phone, in: Digital Soil Morphometrics, Progress in Soil Science Serie, edited by: Hartemink, A. E. and Minasny, B., Springer, 89–110, https://doi.org/10.1007/978-3-319-28295-4_7, 2016. a
Araujo, S. R., Soderstrom, M., Eriksson, J., Isendahl, C., Stenborg, P., and Dematte, J. A. M.: Determining soil properties in Amazonian Dark Earths by reflectance spectroscopy, Geoderma, 237, 308–317, https://doi.org/10.1016/j.geoderma.2014.09.014, 2015. a, b
Australian Government: Carbon Credits (Carbon Farming Initiative) Act 2011, https://www.legislation.gov.au/Details/C2015C00012 (last access: 6 December 2017), 2011. a
Australian Government: Carbon Credits (Carbon Farming Initiative) (Sequestering Carbon in Soils in Grazing Systems) Methodology Determination, https://www.legislation.gov.au/Details/F2015C00582 (last access: 6 December 2017), 2014. a, b, c
Australian Government: Carbon Credits (Carbon Farming Initiative – Estimating Sequestration of Carbon in Soil Using Default Values) Methodology Determination, https://www.legislation.gov.au/Details/F2016C00263 (last access: 6 December 2017), 2015. a, b
Australian Government: Carbon Credits (Carbon Farming Initiative – Measurement of Soil Carbon Sequestration in Agricultural Systems) Methodology Determination 2018, https://www.legislation.gov.au/Details/F2018L00089 (last access: 6 December 2017), 2018. a, b, c
Batjes, N. H.: Total carbon and nitrogen in the soils of the world, Eur. J. Soil Sci., 47, 151–163, 1996. a
Batjes, N. J. and van Wesemael, B.: Measuring and monitoring soil carbon, in: Soil Carbon: Science, Management and Policy for Multiple Benefits, edited by: Banwart, S. A., Noellemeyer, E., and Milne, E., CABI, Wallingford, UK, 188–201, 2015. a, b
Bel'kov, M. V., Burakov, V. S., Kiris, V. V., Kononov, V. A., Raikov, S. N., Reshetnikov, V. N., and Tarasenkoa, N. V.: Determination of carbon in soil by laser spectral analysis, J. Appl. Spectrosc., 75, 275–282, https://doi.org/10.1007/s10812-008-9044-3, 2008. a
Bellon-Maurel, V. and McBratney, A. B.: Near-infrared (NIR) and mid-infrared (MIR) spectroscopic techniques for assessing the amount of carbon stock in soils – Critical review and research perspectives, Soil Biol. Biochem., 43, 1398–1410, https://doi.org/10.1016/j.soilbio.2011.02.019, 2011. a, b
Bellon-Maurel, V., Fernandez-Ahumada, E., Palagos, B., Roger, J. M., and McBratney, A.: Critical review of chemometric indicators commonly used for assessing the quality of the prediction of soil attributes by NIR spectroscopy, Trends Anal. Chem., 29, 1073–1081, https://doi.org/10.1016/j.trac.2010.05.006, 2010. a
Bertuzzi, P., Bruckler, L., Gabilly, Y., and Gaudu, J. C.: Calibration, field-testing, and error analysis of a gamma-ray probe for in situ measurement of dry bulk density, Soil Sci., 144, 425–436, 1987. a
Bispo, A., Andersen, L., Angers, D. A., Bernoux, M., Brossard, M., Cécillon, L., Comans, R. N. J., Harmsen, J., Jonassen, K., Lamé, F., Lhuillery, C., Maly, S., Martin, E., Mcelnea, A. E., Sakai, H., Watabe, Y., and Eglin, T. K.: Accounting for Carbon Stocks in Soils and Measuring GHGs Emission Fluxes from Soils: Do We Have the Necessary Standards?, Front. Environ. Sci., 5, 41, https://doi.org/10.3389/fenvs.2017.00041, 2017. a
Blake, G. and Hartge, K.: Bulk density, Soil Science Society of America, Madison, Wisconsin, USA, 363—375, 1986. a, b
Bornemann, L., Welp, G., and Amelung, W.: Particulate organic matter at the field scale: rapid acquisition using mid-infrared spectroscopy, Soil Sci. Soc. Am. J., 74, 1147–1156, https://doi.org/10.2136/sssaj2009.0195, 2010. a
Brakensiek, D. L. and Rawls, W. J.: Soil containing rock fragments – effects on infiltration, Catena, 23, 99–110, https://doi.org/10.1016/0341-8162(94)90056-6, 1994. a
Bricklemyer, R. S., Brown, D. J., Barefield, J. E., and Clegg, S. M.: Intact soil core total, inorganic, and organic carbon measurement using laser-induced breakdown spectroscopy, Soil Sci. Soc. Am. J., 75, 1006–1018, https://doi.org/10.2136/sssaj2009.0244, 2011. a, b, c
Brown, D. J., Shepherd, K. D., Walsh, M. G., Mays, M. D., and Reinsch, T. G.: Global soil characterization with VNIR diffuse reflectance spectroscopy, Geoderma, 132, 273–290, https://doi.org/10.1016/j.geoderma.2005.04.025, 2006. a
Brus, D. J. and DeGruijter, J. J.: Design-based versus model-based estimates of spatial means: Theory and application in environmental soil science, Environmetrics, 4, 123–152, https://doi.org/10.1002/env.3170040202, 1993. a
Brus, D. J. and de Gruijter, J. J.: Random sampling for geostatistical modelling? Choosing between design-based and model-based sampling strategies for soil (with discussion), Geoderma, 80, 1–44, 1997. a
C'assaro, F., Tominaga, T., Bacchi, O., Reichardt, K., Oliveira, J., and Timm, L.: Improved laboratory calibration of a single-probe surface gamma-neutron gauge, Austr. J. Soil Res., 38, 937–946, https://doi.org/10.1071/SR99107, 2000. a
Chambers, A., Lal, R., and Paustian, K.: Soil carbon sequestration potential of US croplands and grasslands: Implementing the 4 per Thousand Initiative, J. Soil Water Conserv., 71, 68A–74A, https://doi.org/10.2489/jswc.71.3.68A, 2016. a
Chatterjee, A., Lal, R., Wielopolski, L., Martin, M. Z., and Ebinger, M. H.: Evaluation of Different Soil Carbon Determination Methods, Crit. Rev. Plant Sci., 28, 164–178, https://doi.org/10.1080/07352680902776556, 2009. a
Chodak, M., Niklinska, M., and Beese, F.: Near-infrared spectroscopy for analysis of chemical and microbiological properties of forest soil organic horizons in a heavy-metal-polluted area, Biol. Fert. Soils, 44, 171–180, https://doi.org/10.1007/s00374-007-0192-z, 2007. a
Christy, C.: Real-time measurement of soil attributes using on-the-go near infrared reflectance spectroscopy, Comput. Electron. Agr., 61, 10–19, 2008. a
Clairotte, M., Grinand, C., Kouakoua, E., Thebault, A., Saby, N. P. A., Bernoux, M., and Barthes, B. G.: National calibration of soil organic carbon concentration using diffuse infrared reflectance spectroscopy, Geoderma, 276, 41–52, https://doi.org/10.1016/j.geoderma.2016.04.021, 2016. a
Cnudde, V. and Boone, M.: High-resolution X-ray computed tomography in geosciences: A review of the current technology and applications, Earth-Sci. Rev., 123, 1–17, https://doi.org/10.1016/j.earscirev.2013.04.003, 2013. a, b
Cole, C. V., Duxbury, J., Freney, J., Heinemeyer, O., Minami, K., Mosier, A., Paustian, K., Rosenberg, N., Sampson, N., Sauerbeck, D., and Zhao, Q.: Global estimates of potential mitigation of greenhouse gas emissions by agriculture, Nutr. Cycl. Agroecosyst., 49, 221–228, https://doi.org/10.1023/a:1009731711346, 1997. a
Conant, R. T. and Paustian, K.: Spatial variability of soil organic carbon in grasslands: implications for detecting change at different scales, Environ. Pollut., 116, S127–S135, https://doi.org/10.1016/s0269-7491(01)00265-2, 2002a. a
Conant, R. T. and Paustian, K.: Spatial variability of soil organic carbon in grasslands: implications for detecting change at different scales, Environ. Pollut., 116, S127–S135, https://doi.org/10.1016/s0269-7491(01)00265-2, 2002b. a
Conforti, M., Matteucci, G., and Buttafuoco, G.: Monitoring soil organic carbon content using Vis-NIR spectroscopy: A case study in southern Italy, Rendiconti Online Societa Geologica Italiana, 42, 38–41, https://doi.org/10.3301/rol.2017.09, 2017. a
Cook, R. and Weisberg, S.: Residuals and Influence in Regression, Chapman and Hall, New York, 1982. a
Cremers, D. A. and Radziemski, L. J.: Handbook of laser-induced breakdown spectroscopy, John Wiley & Sons, Chichester, UK, 2006. a
Cremers, D. A., Ebinger, M. H., Breshears, D. D., Unkefer, P. J., Kammerdiener, S. A., Ferris, M. J., Catlett, K. M., and Brown, J. R.: Measuring total soil carbon with laser-induced breakdown spectroscopy (LIBS), J. Environ. Qual., 30, 2202–2206, 2001. a, b
Cunningham, R. L. and Matelski, R. P.: Bulk density measurements on certain soils high in coarse fragments, Soil Sci. Soc. Am. Proc., 32, 109–111, 1968. a
Da Silva, R. M., Milori, D., Ferreira, E. C., Ferreira, E. J., Krug, F. J., and Martin-Neto, L.: Total carbon measurement in whole tropical soil sample, Spectrochim. Ac. Pt. B, 63, 1221–1224, https://doi.org/10.1016/j.sab.2008.09.003, 2008. a
de Gruijter, J. J., Brus, D., Bierkens, M., and Knotters, M.: Sampling for Natural Resource Monitoring, Springer, Berlin, 2006. a, b
Don, A., Schumacher, J., Scherer-Lorenzen, M., Scholten, T., and Schulze, E.-D.: Spatial and vertical variation of soil carbon at two grassland sites – Implications for measuring soil carbon stocks, Geoderma, 141, 272–282, https://doi.org/10.1016/j.geoderma.2007.06.003, 2007. a
Ebinger, M. H., Norfleet, M. L., Breshears, D. D., Cremers, D. A., Ferris, M. J., Unkefer, P. J., Lamb, M. S., Goddard, K. L., and Meyer, C. W.: Extending the applicability of laser-induced breakdown spectroscopy for total soil carbon measurement, Soil Sci. Soc. Am. J., 67, 1616–1619, 2003. a, b
Ellert, B., Janzen, H., and McConkey, B.: Measuring and comparing soil carbon storage, Lewis Publishers, Boca Raton, 131–146, 2001. a, b, c
Fan, J. L., McConkey, B., Wang, H., and Janzen, H.: Root distribution by depth for temperate agricultural crops, Field Crops Res., 189, 68–74, https://doi.org/10.1016/j.fcr.2016.02.013, 2016. a
Fontan, J. M., Calvache, S., Lopez-Bellido, R. J., and Lopez-Bellido, L.: Soil carbon measurement in clods and sieved samples in a Mediterranean Vertisol by Visible and Near-Infrared Reflectance Spectroscopy, Geoderma, 156, 93–98, https://doi.org/10.1016/j.geoderma.2010.02.001, 2010. a
Fouinat, L., Sabatier, P., Poulenard, J., Reyss, J. L., Montet, X., and Arnaud, F.: A new CT scan methodology to characterize a small aggregation gravel clast contained in a soft sediment matrix, Earth Surf. Dynam., 5, 199–209, https://doi.org/10.5194/esurf-5-199-2017, 2017. a, b
Gifford, R. M. and Roderick, M. L.: Soil carbon stocks and bulk density: spatial or cumulative mass coordinates as a basis of expression?, Global Change Biol., 9, 1507–1514, https://doi.org/10.1046/j.1365-2486.2003.00677.x, 2003. a
Guerrero, C., Zornoza, R., Gomez, I., and Mataix-Beneyto, J.: Spiking of NIR regional models using samples from target sites: Effect of model size on prediction accuracy, Geoderma, 158, 66–77, https://doi.org/10.1016/j.geoderma.2009.12.021, 2010. a
Guerrero, C., Stenberg, B., Wetterlind, J., Viscarra Rossel, R. A., Maestre, F. T., Mouazen, A. M., Zornoza, R., Ruiz-Sinoga, J. D., and Kuang, B.: Assessment of soil organic carbon at local scale with spiked NIR calibrations: effects of selection and extra-weighting on the spiking subset, Eur. J. Soil Science, 65, 248–263, https://doi.org/10.1111/ejss.12129, 2014a. a, b
Guerrero, C., Stenberg, B., Wetterlind, J., Viscarra Rossel, R. A., Maestre, F. T., Mouazen, A. M., Zornoza, R., Ruiz-Sinoga, J. D., and Kuang, B.: Assessment of soil organic carbon at local scale with spiked NIR calibrations: effects of selection and extra-weighting on the spiking subset, Eur. J. Soil Sci., 65, 248–263, https://doi.org/10.1111/ejss.12129, 2014b. a
Harmon, R. S., De Lucia, F. C., Miziolek, A. W., McNesby, K. L., Walters, R. ., and French, P. D.: Laser-induced breakdown spectroscopy (LIBS) – an emerging field-portable sensor technology for real-time, in-situ geochemical and environmental analysis, Geochem.-Explor. Environ. Anal., 5, 21–28, https://doi.org/10.1144/1467-7873/03-059, 2005. a
Hirmas, D. R. and Furquim, S. A. C.: Simple Modification of the Clod Method for Determining Bulk Density of Very Gravelly Soils, Commun. Soil Sci. Plant Anal., 37, 899–906, https://doi.org/10.1080/00103620600588579, 2006. a
Holmes, K. W., Wherrett, A., Keating, A., and Murphy, D. V.: Meeting bulk density sampling requirements efficiently to estimate soil carbon stocks, Soil Res., 49, 680–695, https://doi.org/10.1071/sr11161, 2011. a, b, c, d
Ibañez Asensio, S., Marques-Mateu, A., Moreno-Ramon, H., and Balasch, S.: Statistical relationships between soil colour and soil attributes in semiarid areas, Biosyst. Eng., 116, 120–129, https://doi.org/10.1016/j.biosystemseng.2013.07.013, 2013. a, b
ICAP: Emissions Trading Worldwide: Status Report 2017, Report, International Carbon Action Partnership (ICAP), Berlin, 2017. a
IPCC: Revised 1996 IPCC Guidelines for National Greenhouse Gas Inventories, Report, Meteorological Office, Bracknell, UK, 1997. a
IPCC: IPCC Guidelines for National Greenhouse Gas Inventories, Report, edited by: Eggleston, S., Buendia, L., Miwa, K., Ngara, T., and Tanabe, K., Intergovernmental Panel on Climate Change (IPCC)/Institute for Global Environmental Strategies (IGES), Hayama, Japan, 2006. a, b
IPCC: Climate change 2007: the physical science basis, in: Contribution of Working Group I to the Fourth Assessment Report of the Intergovernmental Panel on Climate Change, Report, Intergovernmental Panel on Climate Change, Cambridge, UK and New York, NY, USA, 2007. a
ITPS: Status of the World's Soil Resources (SWSR) – Main Report, Report, Intergovernmental Technical Panel on Soils (ITPS), Rome, Italy, 2015. a
Izaurralde, R. C., Rice, C. W., Wielopolski, L., Ebinger, M. H., Reeves, J. B. I., Thomson, A. M., Harris, R., Francis, B., Mitra, S., Rappaport, A. G., Etchevers, J. D., Sayre, K. D., Govaerts, B., and McCarty, G. W.: Evaluation of three field-based methods for quantifying soil carbon, Plos One, 8, e55560, https://doi.org/10.1371/journal.pone.0055560, 2013. a, b, c, d
Ji, W., Viscarra Rossel, R. A., and Shi, Z.: Accounting for the effects of water and the environment on proximally sensed vis-NIR soil spectra and their calibrations, Eur. J. Soil Sci., 66, 555–565, https://doi.org/10.1111/ejss.12239, 2015. a, b
Kennard, R. W. and Stone, L. A.: Computer Aided Design of Experiments, Technometrics, 11, 137–148, https://doi.org/10.2307/1266770, 1969. a
Knadel, M., Gislum, R., Hermansen, C., Peng, Y., Moldrup, P., de Jonge, L. W., and Greve, M. H.: Comparing predictive ability of laser-induced breakdown spectroscopy to visible near-infrared spectroscopy for soil property determination, Biosyst. Eng., 156, 157–172, https://doi.org/10.1016/j.biosystemseng.2017.01.007, 2017. a
Kuang, B., Mahmood, H. S., Quraishi, M. Z., Hoogmoed, W. B., Mouazen, A. M., and van Henten, E. J.: Sensing soil properties in the laboratory, in situ, and on-line, Adv.n Agron., 114, 155–223, https://doi.org/10.1016/B978-0-12-394275-3.00003-1, 2012. a
Lal, R.: Soil carbon sequestration to mitigate climate change, Geoderma, 123, 1–22, https://doi.org/10.1016/j.geoderma.2004.01.032, 2004. a
Lal, R.: Soil carbon management and climate change, Carbon Manage., 4, 439–462, https://doi.org/10.4155/cmt.13.31, 2013. a
Lal, R.: Beyond COP21: Potential and challenges of the `4 per Thousand' initiative, J. Soil Water Conserv., 71, 20A–25A, https://doi.org/10.2489/jswc.71.1.20A, 2016a. a, b
Lal, R.: Soil health and carbon management, Food Energy Secur., 5, 212–222, https://doi.org/10.1002/fes3.96, 2016b. a
Le Guillou, F., Wetterlind, W., Viscarra Rossel, R. A., Hicks, W., Grundy, M., and Tuomi, S.: How does grinding affect the mid-infrared spectra of soil and their multivariate calibrations to texture and organic carbon?, Soil Res., 53, 913–921, https://doi.org/10.1071/sr15019, 2015. a
Lee, J., Hopmans, J. W., Rolston, D. E., Baer, S. G., and Six, J.: Determining soil carbon stock changes: Simple bulk density corrections fail, Agr. Ecosyst. Environ., 134, 251–256, https://doi.org/10.1016/j.agee.2009.07.006, 2009. a
Liles, G. C., Beaudette, D. E., O'Geen, A. T., and Horwath, W. R.: Developing predictive soil C models for soils using quantitative color measurements, Soil Sci. Soc. Am. J., 77, 2173–2181, https://doi.org/10.2136/sssaj2013.02.0057, 2013. a, b
Lin, L. I.-K.: A Concordance Correlation Coefficient to Evaluate Reproducibility, Biometrics, 45, 255–268, 1989. a
Lobsey, C. R. and Viscarra Rossel, R. A.: Sensing of soil bulk density for more accurate carbon accounting, Eur. J. Soil Sci., 67, 504–513, https://doi.org/10.1111/ejss.12355, 2016. a, b, c, d, e, f, g, h
Lobsey, C. R., Viscarra Rossel, R. A., Roudier, P., and Hedley, C. B.: RS-LOCAL data-mines information from large spectral libraries to improve local calibrations, Eur. J. Soil Sci., 68, 840–852, https://doi.org/10.1111/ejss.12490, 2017. a
Lopes, R. T., Bessa, A. P., Braz, D., and de Jesus, E. F. O.: Neutron computerized tomography in compacted soil, Appl. Radiat. Isotop., 50, 451–458, https://doi.org/10.1016/s0969-8043(98)00081-5, 1999. a
Lorenz, K. and Lal, R.: The depth distribution of soil organic carbon in relation to land use and management and the potential of carbon sequestration in subsoil horizons, Adv. Agron., 88, 35–66, https://doi.org/10.1016/s0065-2113(05)88002-2, 2005. a
Machmuller, M. B., Kramer, M. G., Cyle, T. K., Hill, N., Hancock, D., and Thompson, A.: Emerging land use practices rapidly increase soil organic matter, Nat. Commun., 6, 6995, https://doi.org/10.1038/ncomms7995, 2015. a
Martens, H. and Naes, T.: Multivariate Calibration, Wiley, New York, 1989. a, b
Martin, P. D., Malley, D. F., Manning, G., and Fuller, L.: Determination of soil organic carbon and nitrogen at the field level using near-infrared spectroscopy, Canadian Journal of Soil Science, 82, 413–422, https://doi.org/10.4141/s01-054, 2002. a
McCarty, G. W. and Reeves, J. B.: Comparison of NFAR infrared and mid infrared diffuse reflectance spectroscopy for field-scale measurement of soil fertility parameters, Soil Sc., 171, 94–102, https://doi.org/10.1097/01.ss.0000187377.84391.54, 2006. a, b
McConkey, B., Haugen-Kozyra, K., and Staley, D.: Prairie soil carbon balance project summary: Soil organic carbon change on direct-seeded farmland in Saskatchewan, in: Proceedings Soils and Crops 2013 Conference, University of Saskatchewan, Saskatoon, SK, Canada, 2013. a
McKenzie, N., Coughlan, K., and Cresswell, H.: Soil Physical Measurement and Interpretation for Land Evaluation, CSIRO Publishing, Collingwood, Victoria, 2002. a, b
Minasny, B., McBratney, A. B., Bellon-Maurel, V., Roger, J.-M., Gobrecht, A., Ferrand, L., and Joalland, S.: Removing the effect of soil moisture from NIR diffuse reflectance spectra for the prediction of soil organic carbon, Geoderma, 167–68, 118–124, https://doi.org/10.1016/j.geoderma.2011.09.008, 2011. a, b
Moreira, C. S., Brunet, D., Verneyre, L., Sa, S. M. O., Galdos, M. V., Cerri, C. C., and Bernoux, M.: Near infrared spectroscopy for soil bulk density assessment, Eur. J. Soil Sci., 60, 785–791, https://doi.org/10.1111/j.1365-2389.2009.01170.x, 2009. a
Muller, R. N. and Hamilton, M. E.: A simple, effective method for determining the bulk density of stony soils, Commun. Soil Sci. Plant Anal., 23, 313–319, https://doi.org/10.1080/00103629209368590, 1992. a
Naes, T., Isaksson, T., and Kowalski, B.: Locally weighted regression and scatter correction for near-infrared reflectance data, Anal. Chem., 62, 664–673, 1990. a
Nduwamungu, C., Ziadi, N., Tremblay, G. F., and Parent, L.-E.: Near-infrared reflectance spectroscopy prediction of soil properties: effects of sample cups and preparation, Soil Sci. Soc. Am. J., 73, 1896–1903, https://doi.org/10.2136/sssaj2008.0213, 2009. a
Nelson, D. and Sommers, L.: Total carbon, organic carbon, and organic matter, Soil Science Society of America and American Society of Agronomy, Madison, 961–1010, 1996. a, b
Ogle, S. M., Breidt, F. J., and Paustian, K.: Agricultural management impacts on soil organic carbon storage under moist and dry climatic conditions of temperate and tropical regions, Biogeochemistry, 72, 87–121, https://doi.org/10.1007/s10533-004-0360-2, 2005. a
Orr, B., Cowie, A., Castillo Sanchez, V., Chasek, P., Crossman, N., Erlewein, A., Louwagie, G., Maron, M., Metternicht, G., Minelli, S., Tengberg, A., Walter, S., and Welton, S.: Scientific Conceptual Framework for Land Degradation Neutrality. A Report of the Science-Policy Interface, Report, United Nations Convention to Combat Desertification (UNCCD), Bonn, Germany, 2017. a
Papritz, A. and Webster, R.: Estimating temporal change in soil monitoring. 1. Statistical theory, European Journal of Soil Science, 46, 1–12, https://doi.org/10.1111/j.1365-2389.1995.tb01808.x, 1995. a
Paustian, K., Lehmann, J., Ogle, S., Reay, D., Robertson, G. P., and Smith, P.: Climate-smart soils, Nature, 532, 49–57, https://doi.org/10.1038/nature17174, 2016. a
Pedrotti, A., Pauletto, E. A., Crestana, S., Holanda, F. S. R., Cruvinel, P. E., and Vaz, C. M. P.: Evaluation of bulk density of Albaqualf soil under different tillage systems using the volumetric ring and computerized tomography methods, Soil Till. Res., 80, 115–123, https://doi.org/10.1016/j.still.2004.03.003, 2005. a
Peng, Y., Knadel, M., Gislum, R., Deng, F., Norgaard, T., de Jonge, L. W., Moldrup, P., and Greve, M. H.: Predicting soil organic carbon at field scale using a national soil spectral library, J. Near Infrared Spectrosc., 21, 213–222, https://doi.org/10.1255/jnirs.1053, 2013. a
Peng, Y., Knadel, M., Gislum, R., Schelde, K., Thomsen, A., and Greve, M. H.: Quantification of SOC and clay content using visible near-infrared reflectance-mid-infrared reflectance spectroscopy with jack-knifing partial least squares regression, Soil Sci., 179, 325–332, https://doi.org/10.1097/ss.0000000000000074, 2014. a, b
Petrovic, A. M., Siebert, J. E., and Rieke, P. E.: Soil bulk density analysis in three dimensions by computed tomographic scanning, Soil Sci. Soc. Am. J., 46, 445–450, 1982. a
Pires, L. F., Rosa, J. A., Pereira, A. B., Arthur, R. C. J., and Bacchi, O. O. S.: Gamma-ray attenuation method as an efficient tool to investigate soil bulk density spatial variability, Ann. Nucl. Energy, 36, 1734–1739, https://doi.org/10.1016/j.anucene.2009.08.016, 2009. a, b, c
Pires, L. F., Borges, J. A. R., Bacchi, O. O. S., and Reichardt, K.: Twenty-five years of computed tomography in soil physics: A literature review of the Brazilian contribution, Soil Till. Res., 110, 197–210, https://doi.org/10.1016/j.still.2010.07.013, 2010. a
Poeplau, C. and Don, A.: Carbon sequestration in agricultural soils via cultivation of cover crops – A meta-analysis, Agr. Ecosyst. Environ., 200, 33–41, https://doi.org/10.1016/j.agee.2014.10.024, 2015. a
Poeplau, C., Vos, C., and Don, A.: Soil organic carbon stocks are systematically overestimated by misuse of the parameters bulk density and rock fragment content, SOIL, 3, 61–66, https://doi.org/10.5194/soil-3-61-2017, 2017. a
Ramirez-Lopez, L., Behrens, T., Schmidt, K., Stevens, A., Dematte, J. A. M., and Scholten, T.: The spectrum-based learner: A new local approach for modeling soil vis-NIR spectra of complex datasets, Geoderma, 195, 268–279, https://doi.org/10.1016/j.geoderma.2012.12.014, 2013. a
Rawitz, E., Etkin, H., and Hazan, A.: Calibration and field testing of a two-probe gamma gauge, Soil Sci. Soc. Am. J., 46, 461–465, 1982. a
Rayment, G. and Lyons, D.: Soil Chemical Methods – Australasia, CSIRO Publishing, Collingwood, Victoria, 2011. a
Reeves, J. B. I. and Smith, D. B.: The potential of mid- and near-infrared diffuse reflectance spectroscopy for determining major- and trace-element concentrations in soils from a geochemical survey of North America, Appl. Geochem., 24, 1472–1481, https://doi.org/10.1016/j.apgeochem.2009.04.017, 2009. a
Reeves, J. B.: Near- versus mid-infrared diffuse reflectance spectroscopy for soil analysis emphasizing carbon and laboratory versus on-site analysis: Where are we and what needs to be done?, Geoderma, 158, 3–14, https://doi.org/10.1016/j.geoderma.2009.04.005, 2010. a
Reeves, J. B., McCarty, G. W., Calderon, F., and Hively, W. D.: Advances in Spectroscopic Methods for Quantifying Soil Carbon, in: Managing Agricultural Greenhouse Gases, edited by: Liebig, M., Franzluebbers, A., and Follett, R., Elsevier Inc., Amsterdam, 345–366, 2012. a, b, c
Rodionov, A., Patzold, S., Welp, G., Pallares, R. C., Damerow, L., and Amelung, W.: Sensing of soil organic carbon using visible and near-infrared spectroscopy at variable moisture and surface roughness, Soil Sci. Soc. Am. J., 78, 949–957, https://doi.org/10.2136/sssaj2013.07.0264, 2014. a, b
Roudier, P., Hedley, C. B., and Ross, C. W.: Prediction of volumetric soil organic carbon from field-moist intact soil cores, Eur. J. Soil Sci., 66, 651–660, https://doi.org/10.1111/ejss.12259, 2015. a, b
Roudier, P., Hedley, C. B., Lobsey, C. R., Viscarra Rossel, R. A., and Leroux, C.: Evaluation of two methods to eliminate the effect of water from soil vis–NIR spectra for predictions of organic carbon, Geoderma, 296, 98–107, https://doi.org/10.1016/j.geoderma.2017.02.014, 2017. a, b
Rousseva, S., Ahuja, L., and Heathman, G.: Use of a surface gamma neutron gauge for in situ measurement of changes in bulk density of the tilled zone, Soil Till. Res., 12, 235–251, 1988. a
Sankey, J. B., Brown, D., Bernard, M., and Lawrence, R.: Comparing local vs. global visible and near-infrared (VisNIR) diffuse reflectance spectroscopy (DRS) calibrations for the prediction of soil clay, organic C and inorganic C, Geoderma, 148, 149–158, 2008a. a
Sankey, J. B., Brown, D. J., Bernard, M. L., and Lawrence, R. L.: Comparing local vs. global visible and near-infrared (VisNIR) diffuse reflectance spectroscopy (DRS) calibrations for the prediction of soil clay, organic C and inorganic C, Geoderma, 148, 149–158, https://doi.org/10.1016/j.geoderma.2008.09.019, 2008b. a, b
Sarkhot, D. V., Grunwald, S., Ge, Y., and Morgan, C. L. S.: Comparison and detection of total and available soil carbon fractions using visible/near infrared diffuse reflectance spectroscopy, Geoderma, 164, 22–32, https://doi.org/10.1016/j.geoderma.2011.05.006, 2011. a
Sauer, T. J. and Logsdon, S. D.: Hydraulic and physical properties of stony soils in a small watershed, Soil Sci. Soc. Am. J., 66, 1947–1956, 2002. a
Savitzky, A. and Golay, M. J. E.: Smoothing and Differentiation of Data by Simplified Least Squares Procedures, Anal. Chem., 36, 1627–1639, https://doi.org/10.1021/ac60214a047, 1964. a
Segnini, A., Vaz, C., Posadas, A., Guastal, M., Lasso, P., Bernardi, A., and Milori, D.: Chapter 12. Comparative assessment of soil bulk density by computerized tomography methods for carbon stock quantification, Wageningen Academic Publishers, Wageningen, the Netherlands, 2014. a
Senesi, G. S. and Senesi, N.: Laser-induced breakdown spectroscopy (LIBS) to measure quantitatively soil carbon with emphasis on soil organic carbon. A review, Analyt. Chim. Ac., 938, 7–17, https://doi.org/10.1016/j.aca.2016.07.039, 2016. a, b
Shenk, J., Westerhaus, M., and Berzaghi, P.: Investigation of a LOCAL calibration procedure for near infrared instruments, J. Near Infrared Spectrosc., 5, 223–232, 1997. a
Shepherd, K. D. and Walsh, M. G.: Development of reflectance spectral libraries for characterization of soil properties, Soil Sci. Soc. Am. J., 66, 988–998, 2002. a, b
Sherman, D. M. and Waite, T. D.: Electronic spectra of Fe3+ oxides and oxyhydroxides in the near infrared to ultraviolet, Am. Mineralog., 70, 1262–1269, 1985. a
Shi, T., Chen, Y., Liu, H., Wang, J., and Wu, G.: Soil organic carbon content estimation with laboratory-based visible-near-infrared reflectance spectroscopy: feature selection, Appl. Spectrosc., 68, 831–837, https://doi.org/10.1366/13-07294, 2014. a
Shi, Z., Ji, W., Viscarra Rossel, R., Chen, S., and Zhou, Y.: Prediction of soil organic matter using a spatially constrained local partial least squares regression and the Chinese vis–NIR spectral library, Eur. J. Soil Sci., 66, 679–687, 2015. a
Smith, P.: How long before a change in soil organic carbon can be detected?, Global Change Biol., 10, 1878–1883, 2004. a
Smith, P., Martino, D., Cai, Z., Gwary, D., Janzen, H., Kumar, P., McCarl, B., Ogle, S., O'Mara, F., Rice, C., Scholes, B., Sirotenko, O., Howden, M., McAllister, T., Pan, G., Romanenkov, V., Schneider, U., Towprayoon, S., Wattenbach, M., and Smith, J.: Greenhouse gas mitigation in agriculture, Philos. T. Roy. Soc. B, 363, 789–813, https://doi.org/10.1098/rstb.2007.2184, 2008. a
Snee, R. D.: Validation of Regression Models: Methods and Examples, Technometrics, 19, 415–428, 1977. a
Soriano-Disla, J. M., Janik, L. J., Viscarra Rossel, R. A., Macdonald, L. M., and McLaughlin, M. J.: The Performance of Visible, Near-, and Mid-Infrared Reflectance Spectroscopy for Prediction of Soil Physical, Chemical, and Biological Properties, Appl. Spectrosc. Rev., 49, 139–186, https://doi.org/10.1080/05704928.2013.811081, 2014. a
Stenberg, B., Viscarra Rossel, R. A., Mouazen, A. M., and Wetterlind, J.: Visible and near infrared spectroscopy in soil science, Adv. Agron., 107, 163–215, https://doi.org/10.1016/s0065-2113(10)07005-7, 2010. a, b
Stevens, A., Nocita, M., Toth, G., Montanarella, L., and van Wesemael, B.: Prediction of Soil Organic Carbon at the European Scale by Visible and Near InfraRed Reflectance Spectroscopy, PLoS ONE, 8, e66409, https://doi.org/10.1371/journal.pone.0066409, 2013. a
Stiglitz, R., Mikhailova, E., Post, C., Schlautman, M., and Sharp, J.: Using an inexpensive color sensor for rapid assessment of soil organic carbon, Geoderma, 286, 98–103, https://doi.org/10.1016/j.geoderma.2016.10.027, 2017. a
Thamo, T. and Pannell, D. J.: Challenges in developing effective policy for soil carbon sequestration: perspectives on additionality, leakage, and permanence, Clim. Policy, 16, 973–992, https://doi.org/10.1080/14693062.2015.1075372, 2016. a
Tiessen, H., Cuevas, E., and Chacon, P.: The role of soil organic matter in sustaining soil fertility, Nature, 371, 783–785, https://doi.org/10.1038/371783a0, 1994. a
Timm, L. C., Pires, L. F., Reichardt, K., Roveratti, R., Oliveira, J. C. M., and Bacchia, O. O. S.: Soil bulk density evaluation by conventional and nuclear methods, Austr. J. Soil Res., 43, 97–103, https://doi.org/10.1071/sr04054, 2005. a, b, c, d
Tranter, G., Minasny, B., McBratney, A. B., Murphy, B., McKenzie, N. J., Grundy, M., and Brough, D.: Building and testing conceptual and empirical models for predicting soil bulk density, Soil Use Manage., 23, 437–443, https://doi.org/10.1111/j.1475-2743.2007.00092.x, 2007. a
Udelhoven, T., Emmerling, C., and Jarmer, T.: Quantitative analysis of soil chemical properties with diffuse reflectance spectrometry and partial least-square regression: A feasibility study, Plant Soil, 251, 319–329, 2003. a
UNCCD: Decision 22/COP.11, Report, United Nations Convention to Combat Desertification (UNCCD), http://www.unccd.int/en/programmes/Science/Monitoring-Assessment/Documents/Decision22-COP11.pdf (last access: 6 December 2017), 2013. a
United Nations: Transforming Our World: The 2030 Agenda for Sustainable Development, Report, United Nations, https://sustainabledevelopment.un.org/content/documents/21252030 Agenda for Sustainable Development web.pdf (last access: 6 December 2017), 2015. a
Vagen, T. G., Shepherd, K. D., and Walsh, M. G.: Sensing landscape level change in soil fertility following deforestation and conversion in the highlands of Madagascar using Vis-NIR spectroscopy, Geoderma, 133, 281–294, https://doi.org/10.1016/j.geoderma.2005.07.014, 2006. a
VandenBygaart, A. J. and Angers, D. A.: Towards accurate measurements of soil organic carbon stock change in agroecosystems, Can. J. Soil Sci., 86, 465–471, 2006. a
Vasques, G., Grunwald, S., and Harris, W.: Spectroscopic models of soil organic carbon in Florida, USA, J. Environ. Qual., 39, 923–934, 2010. a
Viscarra Rossel, R.: Robust modelling of soil diffuse reflectance spectra by bagging-partial least squares regression, J. Near Infrared Spectrosc., 15, 39–47, https://doi.org/10.1255/jnirs.694, 2007. a
Viscarra Rossel, R. and Lobsey, C.: A new method for rapid measurements of gravel for soil organic carbon accounting, Report prepared for Department of the Environment and Energy, Report, CSIRO Land and Water, Canberra, Australia, 2017. a, b, c, d, e, f
Viscarra Rossel, R. and McBratney, A. B.: Soil chemical analytical accuracy and costs: Implications from precision agriculture, Aust. J. Exp. Agricult., 38, 765–775, 1998. a
Viscarra Rossel, R. and Webster, R.: Predicting soil properties from the Australian soil visible–near infrared spectroscopic database, Eur. J. Soil Sci., 63, 848–860, https://doi.org/10.1111/j.1365-2389.2012.01495.x, 2012. a, b, c
Viscarra Rossel, R., Minasny, B., Roudier, P., and McBratney, A.: Colour space models for soil science, Geoderma, 133, 320–337, https://doi.org/10.1016/j.geoderma.2005.07.017, 2006a. a
Viscarra Rossel, R., Walvoort, D. J. J., McBratney, A. B., Janik, L. J., and Skjemstad, J. O.: Visible, near infrared, mid infrared or combined diffuse reflectance spectroscopy for simultaneous assessment of various soil properties, Geoderma, 131, 59–75, https://doi.org/10.1016/j.geoderma.2005.03.007, 2006b. a, b
Viscarra Rossel, R., Fouad, Y., and Walter, C.: Using a digital camera to measure soil organic carbon and iron contents, Biosyst. Eng., 100, 149–159, https://doi.org/10.1016/j.biosystemseng.2008.02.007, 2008a. a, b
Viscarra Rossel, R., Jeon, Y., Odeh, I., and McBratney, A.: Using a legacy soil sample to develop a mid-IR spectral library, Soil Res., 46, 1–16, https://doi.org/10.1071/SR07099, 2008b. a
Viscarra Rossel, R., Cattle, S., Ortega, A., and Fouad, Y.: In situ measurements of soil colour, mineral composition and clay content by vis-NIR spectroscopy, Geoderma, 150, 253–266, https://doi.org/10.1016/j.geoderma.2009.01.025,2009. a
Viscarra Rossel, R., Adamchuk, V. I., Sudduth, K. A., McKenzie, N. J., and Lobsey, C.: Proximal soil sensing: An effective approach for soil measurements in space and time, Adv. Agron., 113, 237–282, https://doi.org/10.1016/b978-0-12-386473-4.00010-5, 2011. a, b
Viscarra Rossel, R., Webster, R., Bui, E. N., and Baldock, J. A.: Baseline map of organic carbon in Australian soil to support national carbon accounting and monitoring under climate change, Global Change Biol., 20, 2953–2970, https://doi.org/10.1111/gcb.12569, 2014. a
Viscarra Rossel, R., Behrens, T., Ben-Dor, E., Brown, D. J., Dematte, J. A. M., Shepherd, K. D., Shi, Z., Stenberg, B., Stevens, A., Adamchuk, V., Aichi, H., Barthes, B. G., Bartholomeus, H. M., Bayer, A. D., Bernoux, M., Bottcher, K., Brodsky, L., Du, C. W., Chappell, A., Fouad, Y., Genot, V., Gomez, C., Grunwald, S., Gubler, A., Guerrero, C., Hedley, C. B., Knadel, M., Morras, H. J. M., Nocita, M., Ramirez-Lopez, L., Roudier, P., Rufasto Campos, E. M., Sanborn, P., Sellitto, V. M., Sudduth, K. A., Rawlins, B. G., Walter, C., Winowiecki, L. A., Hong, S. Y., and Ji, W.: A global spectral library to characterize the world's soil, Earth-Sci. Rev., 155, 198–230, 2016a. a, b, c, d
Viscarra Rossel, R., Brus, D. J., Lobsey, C., Shi, Z., and McLachlan, G.: Baseline estimates of soil organic carbon by proximal sensing: Comparing design-based, model-assisted and model-based inference, Geoderma, 265, 152–163, https://doi.org/10.1016/j.geoderma.2015.11.016, 2016b. a, b, c
Viscarra Rossel, R., Lobsey, C. R., Sharman, C., Flick, P., and McLachlan, G.: Novel Proximal Sensing for Monitoring Soil Organic C Stocks and Condition, Environ. Sci. Technol., 51, 5630–5641, https://doi.org/10.1021/acs.est.7b00889, 2017. a, b, c
Viscarra Rossel, R. A. and Brus, D. J.: The cost-efficiency and reliability of two methods for soil organic C accounting, Land Degrad. Dev., 29, 506–520, 2018. a, b
Vohland, M. and Emmerling, C.: Determination of total soil organic C and hot water-extractable C from VIS-NIR soil reflectance with partial least squares regression and spectral feature selection techniques, Eur. J. Soil Sci., 62, 598–606, https://doi.org/10.1111/j.1365-2389.2011.01369.x, 2011. a
Wendt, J. W. and Hauser, S.: An equivalent soil mass procedure for monitoring soil organic carbon in multiple soil layers, Eur. J. Soil Sci., 64, 58–65, https://doi.org/10.1111/ejss.12002, 2013. a, b
Wetterlind, J. and Stenberg, B.: Near-infrared spectroscopy for within-field soil characterization: small local calibrations compared with national libraries spiked with local samples, Eur. J. Soil Sci., 61, 823–843, https://doi.org/10.1111/j.1365-2389.2010.01283.x, 2010. a
Wetterlind, J., Piikki, K., Stenberg, B., and Söderström, M.: Exploring the predictability of soil texture and organic matter content with a commercial integrated soil profiling tool, Eur. J. Soil Sci., 66, 631–638, https://doi.org/10.1111/ejss.12228, 2015. a
Wielopolski, L., Hendrey, G., Johnsen, K. H., Mitra, S., Prior, S. A., Rogers, H. H., and Torbert, H. A.: Nondestructive system for analyzing carbon in the soil, Soil Sci. Soc. Am. J., 72, 1269–1277, https://doi.org/10.2136/sssaj2007.0177, 2008. a
Wielopolski, L., Chatterjee, A., Mitra, S., and Lal, R.: In situ determination of soil carbon pool by inelastic neutron scattering: Comparison with dry combustion, Geoderma, 160, 394–399, https://doi.org/10.1016/j.geoderma.2010.10.009, 2011. a
Williams, P. and Norris, K.: Near-infrared Technology: In the Agricultural and Food Industries, 2nd Edn., American Association of Cereal Chemists, St. Paul, MN, USA, 2001. a, b
Yang, H., Kuang, B., and Mouazen, A. M.: Quantitative analysis of soil nitrogen and carbon at a farm scale using visible and near infrared spectroscopy coupled with wavelength reduction, Eur. J. Soil Sci., 63, 410–420, https://doi.org/10.1111/j.1365-2389.2012.01443.x, 2012. a, b
- Abstract
- Introduction
- Soil organic carbon accounting
- Soil sampling, measurement, and the estimation of soil organic C stocks
- Sensors for soil organic C accounting
- Developing a soil organic C accounting methodology with proximal sensing
- Final remarks
- Data availability
- Author contributions
- Competing interests
- Special issue statement
- Acknowledgements
- References
- Abstract
- Introduction
- Soil organic carbon accounting
- Soil sampling, measurement, and the estimation of soil organic C stocks
- Sensors for soil organic C accounting
- Developing a soil organic C accounting methodology with proximal sensing
- Final remarks
- Data availability
- Author contributions
- Competing interests
- Special issue statement
- Acknowledgements
- References